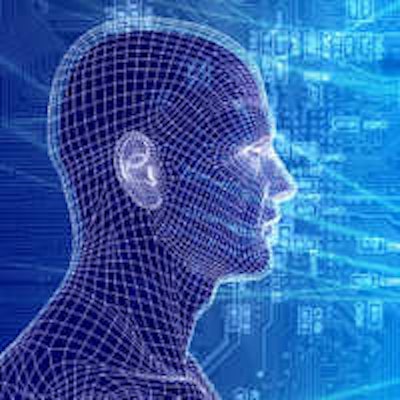
Whilst artificial intelligence (AI) is unlikely to replace radiologists any time soon, a new breed of software applications based on machine learning is poised to take on many of their tedious, repetitive, and time-consuming tasks -- improving productivity and freeing up more time to focus on value-added activities.
In most countries, radiologists are already operating at, or near, capacity; any further gains in efficiency is likely to be derived from the use of "intelligent" workflow software tools. Furthermore, radiology is evolving from a largely descriptive reporting model to a more quantitative discipline, placing added demands on radiologists. As a result, the need for workflow efficiency has never been greater. It's time to cut through the AI hyperbole and take advantage of the many benefits that machine learning is bringing to radiology.
There is a growing array of intelligent image analysis products that automate various stages of the imaging diagnosis workflow. Whilst early generation computer-aided detection (CAD) products largely failed to meet expectations, the application of advanced machine-learning techniques such as deep learning will, in part, enable CAD products to evolve from purely detection systems to more advanced decision-support tools.
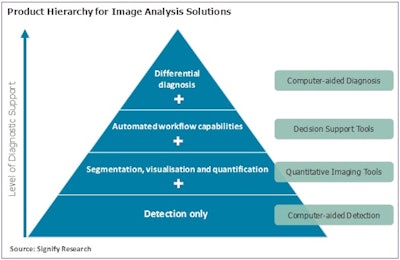
Numbered days for qualitative reports
There is increasing interest and research activity in the field of quantitative imaging -- also known as radiomics -- which requires accurate measurements of imaging biomarkers as indications of disease characteristics. When done manually by a radiologist, this is a time-consuming and often inaccurate process. Not only are quantitative imaging software tools more efficient, the results are more accurate, objective, and repeatable. These tools (e.g., coronary artery calcium scoring, lung density analysis, etc.) have been available for many years and are typically sold as applications for advanced visualization systems.
There is already a sizeable market (see chart below) for quantitative imaging tools -- worth nearly $200 million U.S. (186 million euros) in 2016. In fact, this is by far the largest product category in the overall market for image analysis software, accounting for nearly three-quarters of the revenues in 2016. Developers of image analysis products are increasingly employing advanced (deep) machine-learning algorithms, such as convolutional neural networks. By feeding deep-learning algorithms with radiologist-annotated images and a "ground-truth," the system automatically learns about the image features, rather than being manually programmed (feature engineering) on what to look for.
As such, deep-learning methods enable algorithms to be developed faster, and that potentially will yield more accurate and repeatable results. However, there is currently a lack of large-scale clinical evidence to prove this. Whilst the promise of improved accuracy remains to be seen, deep learning will undoubtedly accelerate product innovation, with new product launches offering innovative features or addressing previously unmet needs.
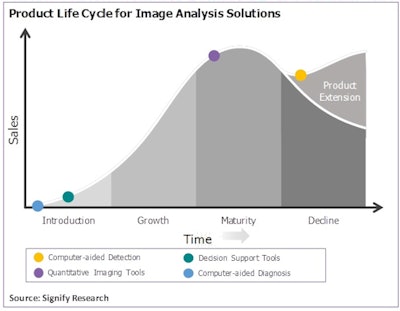
More than imaging data
Decision-support tools provide the same functionalities as quantitative imaging tools, alongside supporting information extracted from an electronic health record (EHR) and other data silos. These reduce the time spent by radiologists navigating these systems in search of useful information. They can present the radiologist with treatment outcomes from similar cases in order to aid with diagnosis and treatment planning. Other capabilities may include automatic population of radiology reports as well as image registration to pinpoint areas of interest in one study that can then be linked to the same points in previous studies.
Today, there are relatively few decision-support tools on the market, but this is likely to be one of the main applications for advanced machine learning in radiology. Several new product launches are expected in the coming years.
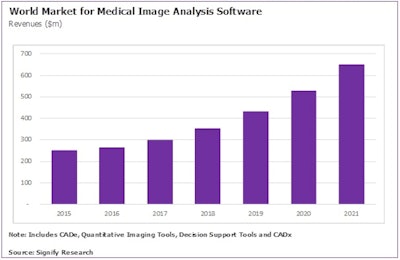
CADx may be closer than you think
Computer-aided diagnosis (CADx) systems are the most advanced class of image analysis software and provide interpretation of medical images, generating, for example a probability score for the presence of cancer. Currently there are no commercially available CADx products for clinical use, but several companies are developing, and in some cases commercializing, image analysis products with differential diagnosis capabilities.
First-generation CADx products are expected to have narrow diagnostic capability for specific body sites, e.g., characterization of breast lesions. This type of CADx system could hit the market before the end of the year, regulatory approval permitting. However, it is likely to be many more years (beyond 2020) before multimodality CADx platforms that offer suggested diagnoses for a broad range of conditions and across multiple body sites become a commercial reality.
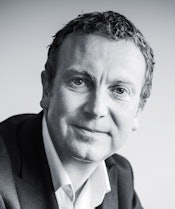
While the first wave of CADx products is on the way, it remains to be seen how these products will be received by radiologists. Many were left underwhelmed by early-generation CAD products and remain skeptical of machine learning's abilities, despite the leap in performance that can be achieved with deep learning over traditional machine learning. Moreover, the "black box" nature of deep learning and the lack of traceability as to how results were obtained could lead to legal implications.
Despite these potential market barriers, deep learning is a truly transformative technology and the longer-term impact on the radiology market should not be underestimated. In the short term, intelligent tools that help radiologists improve their productivity are expected to see the fastest uptake. At this early stage, the prospects for CADx are hard to predict, but the expectation is that the initial market barriers identified above will eventually be overcome. It's more a question of when, not if, machine learning will be routinely used in imaging diagnosis. That day may be sooner than many expect.
This topic and many more are explored in the Signify Research report, "Machine Learning in Medical Imaging -- 2017 Edition."
Simon Harris is managing director and principal analyst at Signify Research, a health technology market intelligence firm based in Cranfield, U.K. He can be reached at [email protected].
The comments and observations expressed herein do not necessarily reflect the opinions of AuntMinnieEurope.com, nor should they be construed as an endorsement or admonishment of any particular vendor, analyst, industry consultant, or consulting group.