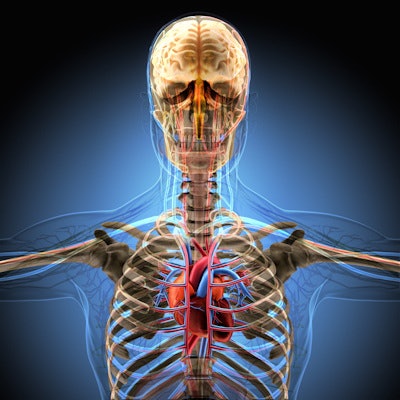
German researchers have developed a method designed to accurately and robustly localize anatomical regions of interest (ROIs) for 3D medical data. They have presented their findings at the International Society for Magnetic Resonance in Medicine (ISMRM) virtual conference.
"Identification of ROIs such as anatomical landmarks, bounding boxes around organs, or the selection of a particular body region in large-cohort imaging data is facilitated by a deep-learning approach," noted Marc Fischer, a PhD candidate at the Department of Radiology, University Hospital Tübingen and Institute of Signal Processing and System Theory, University of Stuttgart, and colleagues in a digital poster presentation at ISMRM.
"We have successfully demonstrated the performance of our network to localize the center of the femur head in MRI and to separate body regions in whole-body CT volumes," they added.
Localizing anatomical ROIs is a common preprocessing step, but it can be complicated for automatic methods. Most machine-learning approaches involve the handcrafting of features to describe differences between ROIs and background, but deep convolutional neural networks (CNNs) overcome this challenge by automatically locating hierarchical feature representations from raw images.
Large epidemiological imaging studies such as the German National Cohort (GNC) require automated pipelines to enable quantitative analyses of thousands of datasets, but the Tübingen team has devised a 3D "deep voting" approach based on recent advances in deep learning. It can locate ROIs, including single points like anatomical landmarks as well as planes to identify region separators within imaging datasets.
How the approach works
The group has used its patch-based neural network for the localization of arbitrary ROIs to perform two tasks: localizing the center of the femoral heads in proton-density-weighted MRI and separating body regions in whole-body CT volumes.
The U-Net-inspired architecture makes use of attentive spatial pyramid (ASP) pooling based on the concepts of ESPNet and CBAM to capture context adaptively. The neural network infers two output volumes, consisting of voxel-wise offset predictions to each ROI and foreground masks around them. Landmarks and region separators are identified as the maximum of the resulting heatmaps.
For landmark localization, the researchers performed a fourfold cross-validation on 80 MR datasets of the GNC (60 training/20 test cases). Proton-density-weighted fast spin-echo images were acquired on a 3-tesla MRI scanner (1.0x1.0x1.0 mm3, matrix size 384x264x160, echo time = 33 ms, repetition time of 1,200 ms, bandwidth = 500Hz/px).
Also, fourfold cross-validation for region separation on 80 contrast-enhanced whole-body CT datasets (60 training/20 test cases) was performed on head, thorax, and abdomen separators with a resolution of 1.6x1.6x5.0 mm3 and a matrix size of 256x256x280.
For the femoral head centers, the mask and the magnitude of the offset vectors were precisely predicted, and users could see them as color-coded overlays. The resulting heatmaps were closely condensed around each center.
For the region separators, the volumes were processed with accurate masks and offset vectors, but there were minor inconsistencies in the prediction. Thus, the resulting aggregated heatmap represented the correct ROI but was not as well condensed.
Quantitative results of the deviations of the located ROIs to the ground-truth positions along relevant dimensions are summarized in the figure below.
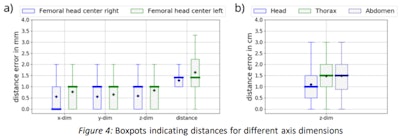
"Regardless of the modality, the approach provides consistent foreground masks and offset predictions. For the landmark localization, excellent results could be achieved, which is a helpful prerequisite for automated segmentation," stated Fischer and colleagues.
"For the CT data with less textural characteristics, a good localization of the region separator could be achieved. In this context, ambiguity of the ground truth must be taken into account, as a single slice has been defined as the separator of two regions," they continued, noting that the future plan is to investigate more complex ROIs such as non-axis aligned region separators.
At the 2019 European Society of Magnetic Resonance in Medicine and Biology congress, the authors gave a presentation about landmark-guided hip segmentation in 3D MRI of a large-scale cohort study.