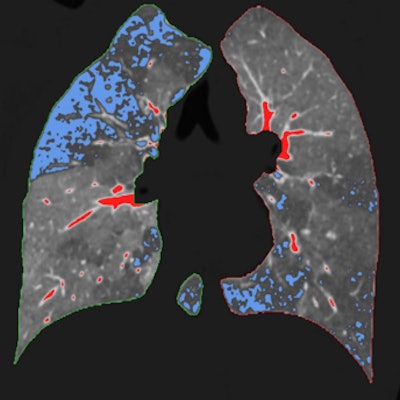
Today's radiologists can use elaborate, computer-supported analysis methods to learn far more from CT data. A study presented at last week's German Radiology Congress (Deutscher Röntgenkongress, RöKo 2018) by the Medical University of Mannheim showed that CT datasets can provide a fairly precise assessment of lung function.
"As radiologists, when we look at CT images we see light and dark areas and we assess the organ using these differences in brightness," said Dr. Joshua Gawlitza, a researcher from the Institute of Clinical Radiology and Nuclear Medicine at Mannheim, who reported the group's findings.
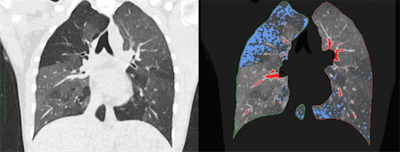
Software programs, which have long been helping radiologists evaluate CT images, usually assess density differences on CT scans and determine average values for the relevant regions.
Pulmonary ventilation & qCT
With the comprehensive datasets of modern CT devices, and in an age where computer capacity no longer represents much of a problem, it is now possible to make completely different assessments. One example of this is quantitative CT (qCT).
"In this process, the software does not just evaluate average densities, but instead analyzes each individual pixel or 'voxel' separately and links them together," Gawlitza explained.
This can prove very interesting in an organ like the lungs, where the qCT evaluates more than two million voxels per lobe, he added. In patients with chronic obstructive pulmonary disease (COPD), for example, the lung is not evenly ventilated. Instead there are areas where the air becomes "trapped" as the patient breathes out, and other areas that work almost normally.
"These badly ventilated areas can be identified with the qCT," Gawlitza noted.
Another example is that of calcification of the coronary vessels, which merely appear as dense deposits in a normal CT with no quantification. The qCT, in contrast, can show the detailed buildup of calcium plaques and their volume, and then quantify the results. This is particularly relevant for the individual risk evaluation of the patient.
Role of neural networks
At the Leipzig congress, Gawlitza and his colleagues reported on a clinical study in which they examined 75 patients with COPD to see if the highly detailed information provided by the qCT could enable them to draw conclusions about lung function.
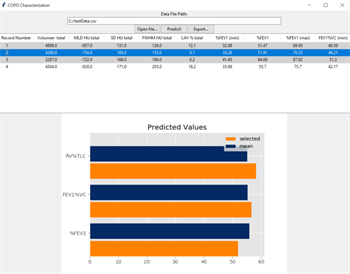
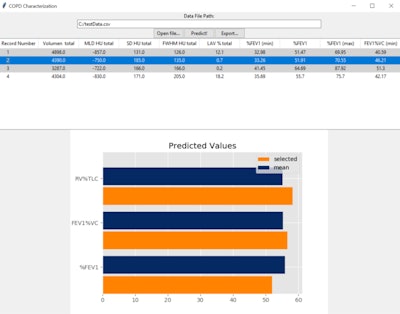
The first prototype of the predictive program from the Medical University of Mannheim, which processes the values collected in the qCT.
"Particularly in clinical research, it can be helpful to determine lung function based on CT data, for example when a patient has CT images, but no lung function data," he continued.
In concrete terms, the Mannheim-based research team fed several qCT parameters -- including lung volume, average lung density, and the percentage of the poorly ventilated lung areas -- into a neural network. They then used PrediCT, a program developed in Mannheim specifically for the qCT assessment of lungs.
"Ultimately the software calculates the lung function based on various models and forms an average. We then compared this with the gold standard, a lung function measurement using body plethysmography," Gawlitza said.
Precision grows with each patient
The preliminary findings, which are based on the CT and body plethysmography datasets of 75 patients, are clear: Only around 10% show any deviation between the lung function parameters, such as FEV1/VC or RV/TLC, calculated by the software and those measured by the gold standard method. The particular appeal of the use of a neural network is the lung function assessments get more accurate over time because the software is capable of learning.
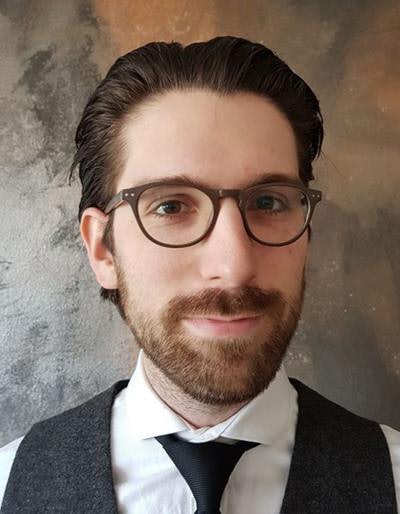
"We are currently examining three to four patients each week as part of the study. Our results will therefore get significantly better," he said.
In the long term, the radiologist believes the combination of qCT and self-learning algorithms will act as a diagnostic tool for a growing number of issues. Among others, he thinks it is highly promising for use in measuring blood circulation, and in cancer treatments to establish the boundaries of tumor tissue. However, Gawlitza does not believe software will replace radiologists any time soon.
"IT solutions like this just mean we can provide the doctors involved with more precise information. At the same time, it will become more and more important to classify this information correctly," he concluded.
Editor's note: This is an edited version of a translation of an article published in German online by the German Radiological Society (Deutsche Röntgengesellschaft, DRG). Translation by Language Connect. To read the original version, visit the DRG website.