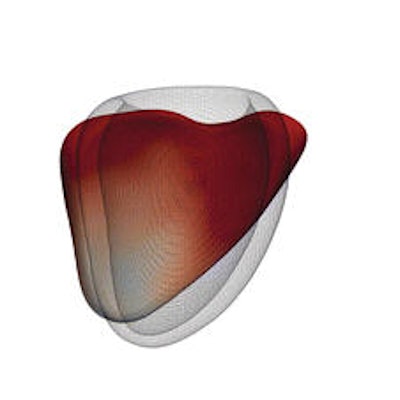
A machine-learning survival model based on 3D cardiac motion predicts outcomes beyond conventional risk factors in patients with pulmonary hypertension, according to a new study from the U.K. and Singapore. Semiautomated analysis of the cine MR images could potentially be used to guide patient management.
The study included 256 patients (mean age 63 ± 17 years, 143 women) with newly diagnosed pulmonary hypertension (PH) and right-sided catheterization, and involved following patients for four years. The investigators used semiautomatic segmentation of short-axis images to create a 3D model of right ventricular (RV) motion. They applied semiautomated principal components analysis to find systolic motion patterns predictive of survival, and found that a loss of effective contraction in the septum and free wall, as well as reduced basal longitudinal motion, were associated with reduced survival (Radiology, 16 January 2017).
"Our study was the first reported use of machine learning to analyze cardiac MR images in order to predict patient survival," noted corresponding author Dr. Declan O'Regan, MRC (Medical Research Council) senior clinician scientist and consultant radiologist at Imperial College London, in an email. "We showed that using a 3D model of cardiac motion added incremental prognostic accuracy compared to conventional risk factors -- including human analysis of cardiac volumes and function."
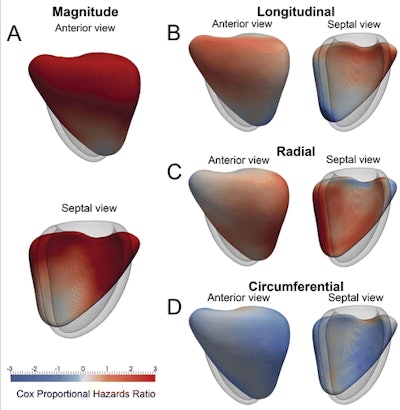
Supervised machine learning of the patterns of cardiac motion showed that survival in patients with pulmonary hypertension PH is predicted by a loss of effective contractile motion in "anatomically distinct but functionally synergistic regions of the right ventricle," explained fellow author Dr. Timothy Dawes and colleagues from the MRC Clinical Sciences Center in London and other centers in London and Singapore. Computational analysis of RV motion in pulmonary hypertension and can be used to stratify risk and show early signs of dysfunction.
PH (defined by a resting mean pulmonary artery pressure of at least 25 mm Hg) can progress quickly with reduced exercise tolerance and dyspnea due to RV hypertrophy, right-sided heart dilation, and ultimately cardiac failure, explained the authors. A wide variety of diagnostic methods have been used to assess outcomes, including invasive hemodynamic data, serum biomarkers, exercise testing, and cardiac imaging.
These markers show that survival is related to functional adaptation of the right ventricle, and RV ejection fraction is a good measure of global function that can be used to predict survival in PH patients, they wrote. At the same time, the right ventricle's complex shape and contraction pattern make it insensitive for assessing cardiac decompensation.
PH patients routinely undergo MRI, which offers a good assessment of cardiac function, but extracting MRI's full predictive value requires choosing only the most meaningful prognostic features.
Untangling functional adaptations
Image analysis coupled with machine learning could explain complex functional adaptations involved, which could be used to predict eventual right-sided heart failure and death, the group wrote. Advances in computational modeling have made such predictions feasible. This study aimed to determine if patient survival and mechanisms of RV failure could be predicted with supervised machine learning of 3D patterns of systolic cardiac motion.
Cardiac MRI was performed using a 1.5-tesla Achieva scanner (Philips Healthcare) and a standard clinical protocol. Ventricular function was assessed by using balanced steady-state free-precession cine images acquired in conventional cardiac short- and long-axis planes with typical parameters.
Semiautomated segmentation of short-axis cine MRI images was used to create a 3D model of right ventricular motion. Supervised principal components analysis was used to identify patterns of systolic motion that best predicted survival. Each marker's performance was tested on all the data, with grouping of significant (0 < 0.05) univariate predictors into three models designed to test the incremental benefit of groups of predictors.
- Model 1 included the clinical, hemodynamic, and functional predictors in univariate prediction.
- Model 2 included those predictors, plus MR volumetry (RV ejection fraction, indexed RV end-systolic volume, indexed RV end-diastolic volume, stroke volume divided by RV end-systolic volume).
- Model 3 included the predictors in models 1, 2, and 3D motion. The models were optimized in the training data and then assessed in the validation data using eightfold cross-validation.
In each cross-validation fold, the group used 224 cases for training, with testing on the remaining 32 cases.
Principal components analysis used a Cox proportional-hazards model for each predictor, then performed feature selection by selecting predictors with coefficients exceeding a threshold. The first component of the reduced data matrix was used as a prognostic marker to predict whether subjects would be alive or dead at censoring, and to fit a Cox proportional-hazards model for subject survival.
Survival was predicted by assessed by using difference in median survival time and the area under the curve with time-dependent receiver operating characteristic analysis for one-year survival, the team wrote.
At the end of follow-up, 36% of patients (93 of 256) had died, and one had undergone lung transplantation. Poor outcomes were predicted by a loss of effective contraction in the septum and free wall, along with reduced basal longitudinal motion.
Outperforming conventional factors
When combined with conventional imaging as well as hemodynamic, functional, and clinical markers, 3D cardiac motion improved survival prediction (area under the receiver operating characteristic curve, 0.73 versus 0.60 without 3D motion analysis (p < 0.001). The motion analysis also offered greater differentiation in terms of difference in median survival time between high-risk and low-risk groups (13.8 versus 10.7 years, respectively; p < 0.001).
Model 3, which included 3D motion, had a significantly higher hazard ratio, area under the ROC curve, and R2 value, along with a greater difference in median survival time than the other models (all p < 0.001).
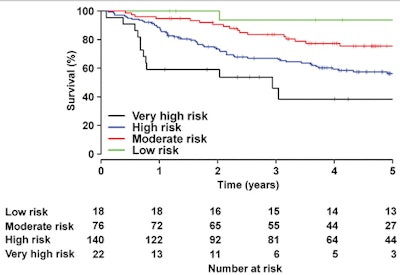
"Supervised machine learning of the patterns of cardiac motion indicates that survival in patients with [pulmonary hypertension] is predicted by a loss of effective contractile motion in anatomically distinct but functionally synergistic regions of the right ventricle," the authors wrote. "A machine-learning survival model that includes cardiac motion has incremental prognostic power when compared with conventional parameters."
Atlas-based analysis of the heart such as used in this study has been used to describe shape variation among asymptomatic adults, identifying the effects of preterm delivery on ventricular geometry, and showing remodeling patterns after a myocardial infarction the authors wrote.
Based on a complex 3D model of cardiac displacement the group applied a machine-learning algorithm to detect recurring patterns in the dataset that enabled the strongest prediction of outcomes. This was used to build a mathematical model depicting cardiac function versus survival.
Previous studies showed that deteriorating RV function is associated with poor outcomes, even when therapy successfully reduces vascular resistance. Imaging can assess systolic function in several ways, for example by global measures of pump function or regional systolic excursion.
The models in this study showed that survival is linked to a combined failure of basal longitudinal shortening and transverse contraction of the septum and free wall, the group wrote. Previous studies have shown the influence of individual components of pump function, while this paper shows their combined influence on outcomes. The modeling also shows the risk associated with adaptations of circumferential function, which tends to increase as global failure develops in pulmonary hypertension.
There is potential for computational simulations not only in risk stratification but also in designing trials for new therapies that have a direct effect on right-ventricular contractility, they wrote. Future work will aim to improve the depth of phenotyping by using time-resolved segmentations throughout the cardiac cycle to model 3D strain tensors.
"The technique could be generalizable to any cause of heart failure if a suitable training population exists," O'Regan told AuntMinnieEurope.com. "We hope to improve accuracy further by using deep-learning algorithms to seamlessly segment the images and make outcome predictions in an end-to-end fashion. Our next steps will be to validate this work on independent patient populations -- and ultimately to assess whether it would lead to better management decisions."