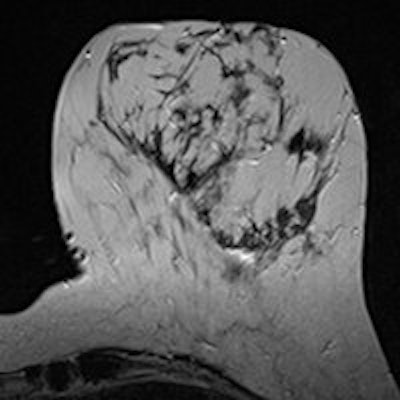
A practical algorithm to classify lesions found in MR mammography (MRM) has been developed by European breast MR heavyweights. A simple decision tree of five criteria reached an accuracy of 88.4% and achieved diagnostic certainty of greater than 95% in more than a third of cases, they found in their study.
Using contrast-enhanced MR mammography, tumors strongly enhance after intravenous injection of contrast medium, but a variety of benign and malignant changes cause enhancement as well. Considering every enhancement as suspicious results in unnecessary biopsies; however, the number of such biopsies can be reduced by an application of diagnostic criteria, according to Dr. Pascal Baltzer from the department of radiology at the Medical University of Vienna in Austria (European Radiology, August 2013, Vol. 23:8, pp. 2051-2060).
"Traditionally, dynamic enhancement patterns describing signal intensity changes over time have been used as diagnostic criteria," wrote Baltzer and his colleagues Dr. Matthias Dietzel from the department of neuroradiology at University Hospital Erlangen in Germany, and Dr. Werner Kaiser from the Institute of Diagnostic and Interventional Radiology at University Hospital Jena in Jena, Germany.
However, the known overlap between dynamic enhancement patterns between these lesion types makes additional criteria "mandatory." Traditionally, these have been mainly morphologic features, but an increased number of diagnostic criteria do not always imply an increased diagnostic accuracy, as different features may convey the same information, they wrote.
"Integration of morphologic and dynamic information into a diagnosis is a complex and experience-dependent task," they added. "Therefore, objective classification schemes integrating morphological criteria are helpful in providing guidance for lesion differentiation."
An intuitive, transparent, and simple classification algorithm is needed, and it was deemed that classification trees were suitable for obtaining more of the information that clinicians desire.
Baltzer and colleagues included a total of 1,084 lesions in standardized MR mammography with subsequent histological verification (648 malignant, 436 benign) in their study. MRI was performed because of unclear findings upon conventional imaging (BI-RADS 0, 3), suspicious conventional findings (BI-RADS 4 and 5), or preoperative staging of biopsy-proven breast cancer (BI-RADS 6) using a 1.5-tesla magnet and a dedicated double breast coil.
Seventeen lesion criteria were assessed by two readers in consensus. Classification analysis was performed using the chi-squared automatic interaction detection (CHAID) method. Results included the probability for malignancy for every descriptor combination in the classification tree.
A classification tree incorporating five lesion descriptors with a depth of three ramifications (1, root sign; 2, delayed enhancement pattern; 3, border, internal enhancement and edema) was calculated. Of all 1,084 lesions, 262 (40.4%) and 106 (24.3%) could be classified as malignant and benign with an accuracy above 95%. Overall diagnostic accuracy was 88.4%.
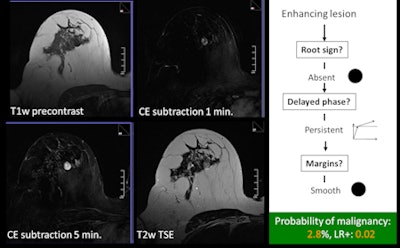
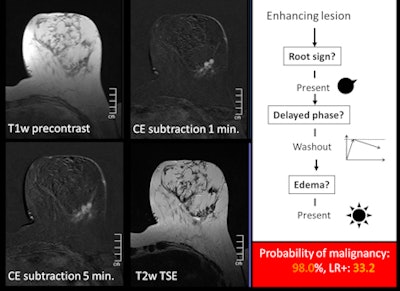
"The result of our study is an easy, intuitive to follow, and valid classification tree for differentiation between benign and malignant enhancing lesions in MRM," the researchers wrote. "Using a simple table or tree scheme, one can classify lesions as being benign or malignant. Such diagnoses can be furthermore automatically classified into being 'definite' or 'most likely' or 'likely to indeterminate' on the basis of the terminal nodes of the CHAID [chi-squared automatic interaction detector] tree and corresponding [positive likelihood ratio] value."
Definite diagnosis with a diagnostic certainty of greater than 95% was found in 34% of all cases. Only a minority of 18.1% of cases showed a classification accuracy of less than 75%, and the reader knows when such a case is diagnosed, Baltzer and colleagues wrote. This means reasonable diagnosis is achieved for the majority 81.9% (888 of 1,084) of lesions.
"Such a statistically validated formal diagnostic decision algorithm providing the user with the diagnostic certainty of his diagnosis has not been described previously," the researchers added. "This may facilitate lesion classification in clinical practice: short-term follow-up and biopsy could be omitted in definitely benign lesions (i.e., high positive likelihood ratio), whereas a follow-up may be warranted in likely benign lesions (positive likelihood ratio clearly below 1)."
The researchers acknowledge biopsy will always be mandatory for malignant lesions in order to decide whether to administer neoadjuvant chemotherapy, but the classification tree may guide clinical decisions in cases of discrepant results between imaging and biopsy results.
A "likely malignant" diagnosis may be explained by mastopathic changes, but a "definite malignant" diagnosis in correlation with the same pathology would make further workup necessary.
"Application of the classification tree does not take extra reading or computational time and may be specifically helpful to nonspecialized readers," they wrote. "Broad application is furthermore facilitated, as only simple dynamic and morphologic features were incorporated, avoiding the necessity for specific protocols or postprocessing software."
The researchers note the positive predictive values given in the terminal nodes of their tree are only valid for the prevalence of malignancy (59.8%) in their patient group as predictive values mathematically depend on pretest probability -- i.e., prevalence. That is why they generally prefer likelihood ratios for all tree nodes as a more appropriate statistical means.
"The reader can thus convert the individual pretest probability of malignancy into a post-test probability," they wrote. "This approach is highly flexible and allows integration of further patient-related data like prior imaging results, family, and personal history."
Limitations
The researchers noted a few limitations of the study:
Only histopathologically verified lesions were considered, potentially biasing the lesion database toward more difficult diagnosis in which invasive diagnosis was requested in the first place.
No quantitative techniques were used. Recommendations for MR mammography include dynamic and T2-weighted protocols, but not diffusion-weighted imaging, dynamic contrast-enhanced MRI, or spectroscopy because the diagnostic value of these techniques has not been clarified yet. Furthermore, results of quantitative MRI techniques critically depend on the acquisition parameters.
The lesion features are not totally congruent with the BI-RADS lexicon because the prospectively populated database was started prior to the publication of the BI-RADS lexicon.
Various interpretation models have been proposed in the past by other researchers. All of them contain delayed-enhancement curve type (persistent, plateau, washout). Border characteristics and internal enhancement pattern seem to be the most important morphologic criteria.
"It is assumed that variability of patient characteristics, diagnostic criteria used, and limited statistical validation is a major reason for the variety of results of interpretation models published so far," the researchers wrote. "Predictive values depend on pretest probability and should thus be considered with care in different clinical contexts. This is why we provide likelihood ratios, which allow one to model individual results."
Baltzer and colleagues have provided a simple and robust classification model that follows an intuitive tree structure reflecting a structured step-by-step diagnostic process for lesion differentiation, they concluded.
"It is based on the largest database published so far in this context, thus ensuring statistical stability," they added. "Every single combination of diagnostic criteria is associated with a specific and relative likelihood of malignancy, and thus provides the reader with an objective tool for making clinical decisions."
In an interview with AuntMinnieEurope.com, Baltzer said the researchers plan to conduct a prospective, multicentric and multinational validation study, but the likelihood ratios provided by the tree could be used for a Bayesian approach on lesion diagnosis.
"That means a probability of malignancy and thus a BI-RADS diagnosis can be derived from the classification tree results combined with the initial clinical and imaging information (Palpable lump? Bloody discharge? Mammographic lesion? etc.)," he stated. "The classification tree is highly open to be combined with additional findings and thus more flexible and more specific than prior classification systems for breast MRI."