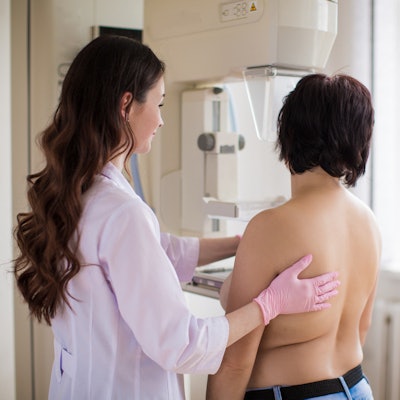
The number of screening exams for colorectal and breast cancer performed at the COVID and Cancer Research Network (CCRN) dropped by nearly 90% in April due to the COVID-19 pandemic, according to research published online on 27 July in JCO Clinical Cancer Informatics.
A team of researchers led by Jack London, PhD, of Thomas Jefferson University in Philadelphia utilized the TriNetX research network platform to analyze de-identified data of over 28 million patients at 20 U.S. healthcare institutions. Using data from the CCRN and also from University Hospitals Plymouth National Health Service (NHS) Trust in the U.K, the researchers compared cancer patient cohorts before COVID (January-April 2019) to after COVID (January-April 2020). They also calculated the number of patients who received cancer screenings.
Change in number of cancer screening tests from 2019 to 2020 by month | ||
Colorectal cancer screening (colonoscopy, CT scans) | Mammography | |
January | 0.7% | -5% |
February | -5.6% | -9.1% |
March | -39.4% | -43.8% |
April | -84.5% | -89.2% |
The researchers also found substantial drop-offs in the number of cancer patients having encounters in April 2020:
- Melanoma: -58.1%
- Prostate cancer: -49.1%
- Breast cancer: -47.7%
- Colorectal cancer: -39.9%
- Lung cancer: -39.1%
- Hematologic cancer: -39.1%
"Trends seen in the CCRN clearly suggest a significant decrease in all cancer-related patient encounters as a result of the pandemic," the authors wrote. "The steep decreases in cancer screening and patients with a new incidence of cancer suggest the possibility of a future increase in patients with later-stage cancer being seen initially as well as an increased demand for cancer screening procedures as delayed tests are rescheduled."