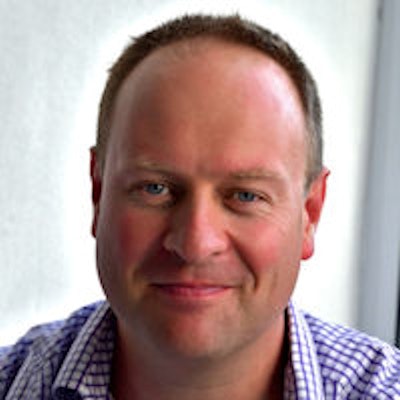
For the past five years, I've been devising a collection of simple rules of radiology -- the uber-truths that are the very essence of our beloved specialty. Here's the final batch of 30 rules for you to absorb and adhere to.
Rule 1: Eminence doesn't equal sense
Confidence wears off slower than skill in the aged radiologist. Grey hair and notoriety doesn't mean infallibility. Often the opposite is the case.
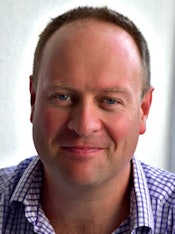
Rule 2: Sloppiness is infectious
People inherently cut corners. Sometimes it is because they work in a bad system. Sometimes it is because they are lazy. Don't just shrug complacently -- do something. The standard you walk past is the standard you accept.
Rule 3: Never assume relationships
An attractive 20-something with a frail octogenarian are usually grandchild and grandparent. But the one time you voice this, you can guarantee they'll be married. Avoid foot-in-mouth disease -- always ask.
Rule 4: "Order comms" must be loathed
First, no radiologist should let themselves be "ordered" to do anything. Second, one-way transmission prevents clinicoradiological discussion. Last, it denies a key perk of the job as there is no physical card to rip up.
Rule 5: Don't blame the "bad apple"
Improvement by removing a "bad apple" from the barrel is a fallacy. It is virtually always a barrel issue. It is just that apples are easier to blame. An enlightened radiologist blames neither: They learn and they improve.
Rule 6: Structured reporting is overblown
Structure in reports is always good, but structured reports aren't always good. Such reports are over-long and mind-numbingly bland -- the antithesis of a readable report. And an unreadable report is a dangerous report.
Rule 7: Beware pixel squeezers
Some radiologists will try to convince you of the significance of tiny isolated abnormalities only a few pixels across. Or worse, minor variations in the shade of faux color. You can safely ignore them; they are making it up.
Rule 8: Embrace productivity
Attempts to improve productivity are normally just harder cracks of the same whip. This achieves nothing apart from burnout. But looking at ways of achieving the same goal more easily and effectively indicates enlightenment.
Rule 9: Be a kind boss
Radiologists can find themselves in leadership positions, often accidentally. Be the boss you would like to have had. You can be decisive, effective, and yet remain kind; whereas inhuman autocrats are toxic, ineffective, and hated.
Rule 10: Embrace diversity
Great departments are staffed by radiologists of varying age, gender, race, culture, and personality. This serves both referrers and patients well. But coherence is vital. It falls flat if you aren't all pulling in the same direction.
Rule 11: Look after yourself
You cannot escape the anxieties that are part of professional life. But when going the extra mile becomes the norm, you are on the road to burnout. A burnt out radiologist is tragic: tragic for themselves, tragic for their patients.
Rule 12: It's all about the patient
It isn't about you, the referrer, the institution, or the health system. It never was and never will be. Everything we do should benefit the patient in front of you. Lose that and we lose everything.
Rule 13: Radiology is now the "Fat Lady"
It used to be said that "case discussion is not over until the pathologist sings." This is no longer true. The radiologists now sing the harmony, the surgeons join in on the chorus, and the pathologists merely hum along. But no one is quite sure what, if anything, they are humming.
Rule 14: Workspaces should be spotless
Nothing says unprofessional more than an untidy desk, a cluttered PACS workstation, or a messy reporting room. It is depressing, demotivating, and distracting. What are you waiting for? Go wash your mug now!
Rule 15: Beware the radiologist that becomes "one of them"
Radiologists occasionally go over to the dark side when they take up management roles. When they say phrases like "going forward," "paradigm shift," or "action plan," you must sever all ties quickly and quietly. They are a positive menace to the department.
Rule 16: Don't wallow in either glory or misery
Like hangovers, neither triumphs nor disasters last forever.
Rule 17: Treat people like people
The frail, elderly patient you accidentally patronize may be a Nobel Prize winner. The patient you assume is deaf and/or demented is usually neither.
Rule 18: Smile
Those who complain the most, accomplish the least. And remember, nobody likes a whinger.
Rule 19: Keep your cool
Losing your temper always makes things worse. Anger reveals weakness of character. Equanimity is hard but worth it.
Rule 20: Don't be too approachable
You don't want to be the one who gets asked to do everything anymore than you want to be the one that everyone slags off as being grumpy and lazy.
Rule 21: Agitation is not an indication
Lack of planning on their part does not constitute an emergency on yours. Let them get away with it and they don't learn the lesson.
Rule 22: Always help the patient
Helping the medical team is helping the patient. The natural tendency is, of course, to say yes. Apply the "grandma test" -- what if it was your grandma? Sometimes helping means saying no -- it might not be in the patient's best interests.
Rule 23: Don't study surrogates
Many radiologists spend hours studying, with great precision, that which does not matter. Cut to the chase; study important primary outcomes, irrespective of how hard it is; anything else is lazy.
Rule 24: Avoid "interesting" cases
They aren't. They've asked everyone else already. And, no, they don't have a clue either.
Rule 25: Beware the "fit 90 year old"
There is no such thing.
Rule 26: Counteract misjudgment
Staff and patients make snap judgments about you. Overcompensate. Dress smarter and behave more professionally than you think you should.
Rule 27: Trust your clinical instincts
Take a brief history and tailor the examination appropriately -- the patient is trying to tell you their diagnosis. However, if your barium enema patient starts Cheyne-Stokes breathing just as you are seeing the cecum properly, you should probably stop.
Rule 28: If you have to fail, fail safely
Struggling is learning. But only to the point that adrenaline turns brown. Recognize your boundaries and approach them cautiously, with the cavalry in the wings.
Rule 29: Take clinical details with a pinch of salt
We were all junior doctors; we know why they write the clinical details they do. We understand that clinical examination is fickle -- the words "shifting dullness" are 100% negatively predictive for ascites on ultrasound. We understand the pressures -- "?SDH" (subdural hemorrhage) on a CT head card merely means the boss's ward round is looming.
Rule 30: Obey the rules
Your reason to knowingly breach the rules isn't good enough. It never will be. It is also forbidden for someone familiar with the rules to knowingly assist another person to breach them.
Dr. Paul McCoubrie is a consultant radiologist at Southmead Hospital in Bristol, U.K.
The comments and observations expressed herein do not necessarily reflect the opinions of AuntMinnieEurope.com, nor should they be construed as an endorsement or admonishment of any particular vendor, analyst, industry consultant, or consulting group.