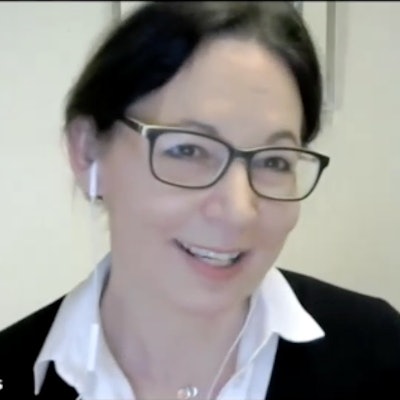
How is artificial intelligence (AI) being used in daily routine? How does it improve workflow and empower researchers and patients? What does the future hold? Prof. Dr. Marion Smits, PhD, the newly elected chair of the European Society of Radiology (ESR) Research Committee, addressed these questions in a webinar on 10 February.
In this Q&A article, Smits speaks about the most exciting areas of AI development in neuroradiology and the topics of special interest to her, such as data sharing. She also describes her goals and priorities in the new ESR role and how she combines research and clinical work as a neuro- and head and neck radiologist, with a particular focus on neuro-oncology in the Brain Tumor Center.
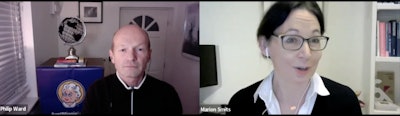
Q: In your work as section chief of neuroradiology at Erasmus MC in Rotterdam, how integral is AI to your clinical practice?
A: We're a really large research site for AI. We always have some form of AI testing, validation, and evaluation going on, and we see a lot of prototype products in a testing context. But we do use some AI for routine clinical practice, mostly for quantification, and more specifically for the Memory Clinic and for the Brain Tumor Center as well.
The Memory Clinic software is commercial, CE marked software that we've been using for years to quantify the volume of brain, brain lobes, and hippocampus and compare that with a reference standard. This AI is really integral to our practice, both for us as radiologists and for discussion in our multidisciplinary team meetings with the neurologist and other treating physicians. We've recently started using automated tumor segmentation at the Brain Tumor Center, using in-house software developed by one of my PhD students in selected cases.
Q: Could you tell us about your research group and current projects involving AI?
A: I have eight PhD students, one postdoctoral researcher, and two assistant professors, and they include clinical radiology researchers, computer scientists, and MR physicists with a very technical background. We've just concluded a project called iGene about the prediction of brain tumor genotypes using AI. Most of the work, if not about the actual coding, is about developing biomarkers that we can then use to build AI tools for neuro-oncology.
Q: In the recent ESR election, you spoke about the need to empower researchers. Does AI have a role here?
I think so. I want to combine research and clinical practice. As a researcher, you don't always have all the skills needed for that combination, and AI can help because it can provide the tools and expertise required for research and it reduces workload.
From a cost-benefit analysis point-of-view, radiologists are a substantial expense, so taking away labor-intensive and time-intensive tasks from us is the obvious first cost that you can reduce with AI. For example, being able to do tumor segmentation automatically is great.
Q: In the election, you also referred to the need to break down barriers between disciplines. Is AI useful in this area?
A: Yes, I think AI can be the driving force there. Disciplines are bound by expertise. I'm an expert in neuroradiology, but AI can now sometimes perform at the same level as experts. For example, our own algorithm was just as good as I was at predicting tumor type, and it was a lot better than some of the nonexpert radiologists. So this means that those boundaries between disciplines will disappear. Not everybody will be happy about that. The important thing here is that it means that boundaries between institutions may also disappear.
AI can empower patients too. Patients will have better access to highly expert diagnostics, whether they go to a university hospital or other types of hospital -- as long as they run the AI tools, they should get the same diagnostic expertise.
I am working on projects where I am trying to get patient input on AI tools so that they can visualize diagnostics. Having access to a lot of information from MRI scans instead of after surgery helps them understand tumor type and prognosis straight out of the scanner, and this can help empower them to make their own decisions, to be in charge of their plan ahead of other interventions that are now necessary to get that same information.
This would be the ideal scenario, but we're not there yet. It will take more time and work.
Q: You're a supporter of open access and data sharing, but with the need for confidentiality, is it always possible to be completely open and transparent?
A: That's the challenge. We need a lot of good quality, diverse data. AI development is driving this trend for data sharing, as are the people working on AI who are willing to share their data, and their source codes. Younger generations are happier to share as well. It's really inspiring to see, and this is opening our eyes.
Bias and quality in AI development is a big topic; a lot of our data is very biased and it is discriminating against certain groups of patients. We need transparency to know how the algorithm has been developed and tested so that we can ensure that this AI tool works for a patient anywhere in the world. At the very least, we need to know who it works for and who it doesn't work for.
While issues of confidentiality remain, there is such a will for data sharing that will overcome the hurdles. We've just published the Erasmus Glioma database that contains over 700 patients' scans and includes clinical details and tumor types. We're already getting many data-sharing requests for this. It's fantastic that so many people will use it to get to better algorithms and better diagnostics for these patients.
Q: Do you have any advice for developers of AI?
A: What's really important is that we talk to each other. It would be great if together we could find out more about the clinical needs and the academic challenges because they don't always overlap.
The other thing we need to collaborate on is "trustworthy AI," allowing insight into decision making and into the certainty of predictions by the algorithm to make the diagnosis. That's something that is sometimes lacking at the moment.
Q: Is the clinical implementation of AI frustratingly slow at times?
A: Yes and no. It's very easy to forget how much progress has been made because as soon as something works you just forget it as it seamlessly fits in. The things that don't work frustrate you, but implementation is really happening and it's moving ahead fast in some areas.
Q: Looking to future clinical applications of AI, which areas excite you most as a neuroradiologist?
A: It's quite mundane really, but I often joke that if I never have to measure a tumor again, I'll be the happiest woman in the world!
This is especially the case in glioma and other brain tumors that are very challenging. It's not the most exciting task, but it would really save the day and leave my brain power for the complex stuff and talking to clinicians about the complicated cases.
Editor's note: You can watch a recording of the AuntMinnieEurope.com and AuntMinnie.com webinar, "AI Trends in 2022: Neuroradiology," on demand via this link. The other two speakers were Prof. Dr. Alexander Radbruch from Bonn, Germany, and Dr. Peter Chang from California. The next AI webinar in this series will take place on 6 April. The series is being produced by Brian Casey, editor in chief of AuntMinnie.com, and sponsored by Bayer.