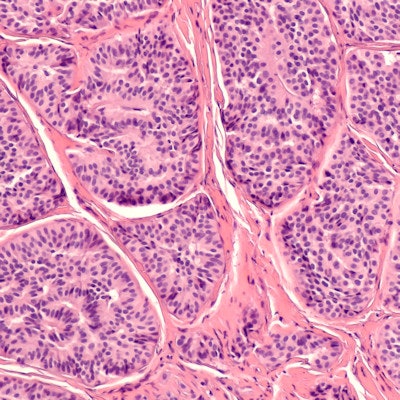
Body composition analysis of routine PET/CT staging exams can be used to opportunistically monitor patients with neuroendocrine tumors of a gastroenteropancreatic origin (GEP-NET), according to research published on 4 August in Scientific Reports.
A team of researchers from University Hospital Essen in Germany found that CT-based body composition analysis by a deep-learning algorithm yielded accurate and stable results when compared with dual-energy x-ray absorptiometry (DEXA) or bioelectrical impedance analysis (BIA) in monitoring these patients.
"[CT-based body composition analysis] implemented as a substitute for these modalities could substantially improve the quality of life for the individual patient alongside the reduction of costs, radiation dose, and consumption of resources in general," wrote co-first authors Dr. Lennard Kroll, Dr. Annie Mathew, Dr. Felix Nensa, and Dr. Harald Lahner.
Currently, DEXA or BIA is used in patients with GEP-NET to analyze fat and muscle composition changes that could indicate whether the cancer is progressing or is responding to treatment. The researchers sought to assess whether their previously developed deep-learning algorithm for CT-based body composition analysis could be employed instead in these patients.
"Cutting out additional radiation dose and up to two additional examinations by employing [body composition analysis] into the diagnostic algorithm would be an improvement to patient care due to its generalizable, time, and resource-efficient workflow compared to non-automated [body composition] software," the authors wrote.
The researchers performed automated body composition analysis on 74 consecutive GEP-NET patients who had received whole-body gallium-68 (Ga-68) DOTATOC PET/CT exams as well as BIA and DEXA scans between February 2019 and October 2021. The deep-learning model was applied to the contrast-enhanced, 5-mm, whole-body CT images for all 74 patients.
The model examined seven volumes for different body compartments and quantified subcutaneous adipose tissue, visceral adipose tissue, intermuscular adipose tissue, epicardial adipose tissue, and paracardial adipose tissue. Muscular and bone tissue volumes were computed to generate a skeletal muscle ratio and body fat ratio.
Overall, the model produced a mean Sørensen-Dice score of 0.93, demonstrating high accuracy, according to the authors. After calculating Pearson's rank correlations (r) and Lin's concordance correlation coefficient (ρC), the researchers found that the automated CT-based body composition analysis also correlated highly with the DEXA and BIA results.
Correlation of automated CT-based body composition algorithm with BIA, DEXA | ||
Correlation with BIA | Correlation with DEXA | |
Correlation of automated CT-based calculation of body fat ratio | r = 0.92, pC = 0.76 | r = 0.95, pC = 0.83 |
Correlation of automated CT-based calculation of skeletal muscle ratio | r = 0.81, pC = 0.49 | n/a |
Pearson correlation coefficient analysis showed that the correlations were all statistically significant (p < 0.001).
Using PET/CT scans to monitor body composition could reduce the need for additional exams while "substantially amplifying measurement in slower progressing cancers such as GEP-NET," according to the researchers.
"The emergence of automated techniques to quantify body composition will allow for rapid and early intervention, especially of high-risk patients," the authors wrote. "The integration of body composition measurement into oncology offers a tremendous promise to help patients with cancer live longer and healthier lives and experience enhanced [quality of life]. These factors should be focused on by future multicenter studies with larger cohorts and correlation to clinical endpoints."