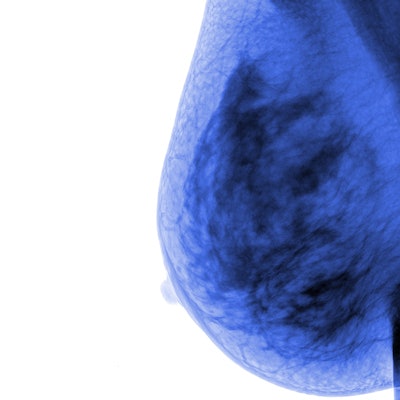
A novel AI tool can accurately detect and characterize microcalcifications on mammography, an Italian study published on 7 November in European Radiology Experimental has found.
Researchers led by Dr. Filippo Pesapane, a radiologist at the European Institute of Oncology (IEO) in Milan, developed three neural networks from a mammogram dataset and found that all three achieved high area under the curve (AUC) values in detecting microcalcifications.
“These findings are not just a testament to the model’s accuracy, but also speak volumes about the potential of in-house, clinically developed AI tools in enhancing diagnostic precision in breast cancer screening,” Pesapane told AuntMinnieEurope.com.
In breast imaging, AI has shown its potential as a clinical assistant to interpreting radiologists. This includes detecting suspicious findings on mammography, predicting breast cancer risk, and assessing microcalcifications.
Pesapane and co-authors sought to develop and test an AI model in-house for localizing and characterizing microcalcifications. To do so, three radiologists annotated a dataset of mammograms using histology-based ground truth. For annotation, the radiologists used a tagging tool (X-RAIS, Laife Reply) to correlate the location of suspicious microcalcifications for each image and the binary classification of benign malignant findings. These were reported by the outcome of the histological investigation.
From there, the team trained three neural networks: AlexNet, ResNet18, and ResNet34. These networks were also validated and tested using an annotated dataset of 1,000 patients and 1,986 mammograms. Of the total, 389 groups of microcalcifications were deemed malignant while 611 were benign.
The team evaluated the neural networks in their ability to detect and classify microcalcifications. It found that overall, AlexNet had the best overall performance of the three neural networks.
Performance of neural networks in assessing breast microcalcifications | |||
---|---|---|---|
ResNet34 | ResNet18 | AlexNet | |
Detection | |||
AUC | 0.98 | 0.98 | 0.98 |
Sensitivity | 0.97 | 0.96 | 0.98 |
Specificity | 0.9 | 0.91 | 0.89 |
Classification | |||
AUC | 0.92 | 0.88 | 0.94 |
Sensitivity | 0.94 | 0.75 | 0.85 |
Specificity | 0.84 | 0.85 | 0.89 |
The researchers also found that AlexNet had the negative predictive value of the three networks in detection (0.94) and classification (0.88).
Pesapane, who is an active member of the editorial advisory board of AuntMinnieEurope.com, said that all in all, these models have the potential to improve the work of breast radiologists, especially in breast cancer screening programs. He noted that by developing these models in-house, the researchers can incorporate rich and diverse datasets, “reflective of the actual population we serve, including varying breast densities and demographic backgrounds.”
“We're looking at a tool honed directly within a clinical setting, designed with patient diversity in mind, a factor I think is crucial for its intended real-world application,” he added.
The full study can be found here.