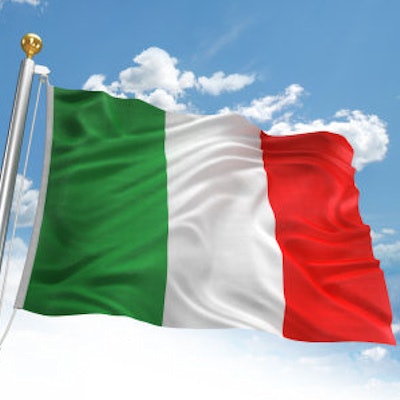
Software based on natural language processing and machine-learning techniques can effectively classify free-text radiology reports, showing potential for improving clinical care and supporting radiology research, according to an Italian study published online on 7 June in Artificial Intelligence in Medicine.
Researchers led by Alfonso Emilio Gerevini, PhD, of the University of Brescia have developed a software system that can automatically analyze and classify chest CT reports in Italian by the nature of the examination (e.g., first or follow-up study), test result, nature of the lesion, site, and lesion type. While the hierarchical classification system still has room for improvement, the authors reported encouraging results during initial testing.
Traditional radiological reporting has produced a vast trove of free-text clinical narratives that could be used for enhancing clinical care and research, but automatic techniques are needed to analyze these reports and make their content effectively available to radiologists in an aggregated form, they noted. In an attempt to help, they developed a machine learning-based system that was trained using chest CT reports annotated based on a particular classification schema by radiologists from their institution. The group also marked the textual evidence for their classification in each report.
To arrive at a classification decision, their machine-learning method utilizes a "cascade of classifiers" both syntactic and semantic in the report. In testing, the system could correctly classify only a little more than half of the three most important features in 68 reports. However, a separate analysis of the system's automatic annotation module found 85% agreement with an expert radiologist, providing evidence it often correctly identifies the parts of the text that are informative for the classification tasks, the researchers said.
The system's ability to identify the most relevant sentences in the report could in the future enable physicians to more easily read and understand a report, according to the researchers. Since the initial research was performed, the researchers have added a level to the classification schema that accounts for follow-up examinations and the site for which the study was recommended. In future plans, they said they intend to expand the dataset to consider different radiology departments and other annotators. New reports annotated by another expert will be included in the experimental datasets in the near future, they said.
In addition to plans for extending the classification scheme to other parts of the body, the researchers will explore the use of additional machine-learning and text-processing techniques such as deep neural networks and more sophisticated document representations for text classification. They've also begun integrating the classification system into their institution's radiology reporting software, which will enable radiologists to obtain a real-time report classification that could be immediately confirmed or modified, according to the researchers.
"The automatic annotation module will also be used for highlighting the relevant sentences of a report in order to improve visualization [of the findings]," they wrote. "We plan to analyze how/if the automatic annotation and classification of new reports helps the radiologists."