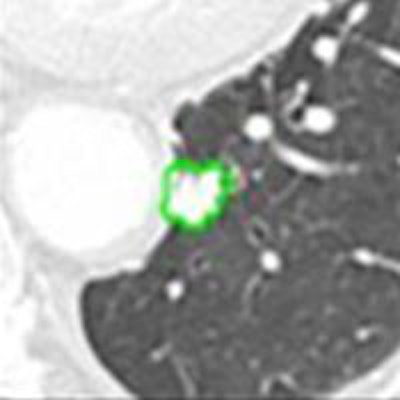
Researchers in the Netherlands have developed a new computer-aided detection (CAD) scheme that can pick up large pulmonary nodules at thoracic CT, plugging a disappointing gap in current CAD technology.
In more than 200 patients with lung nodules larger than 10 mm, the system achieved sensitivity of more than 95% or 85%, depending on the number of false-positives allowed (7.5 or one per case, respectively), the study team reported.
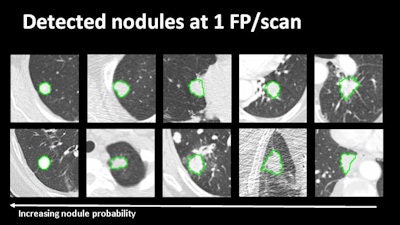
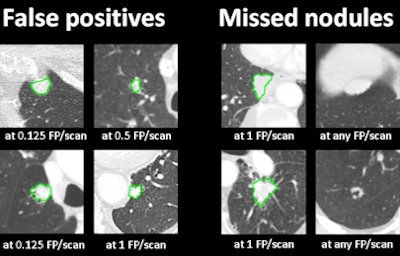
Lung CAD systems routinely miss nodules larger than 10 mm because most of the time the algorithms have been trained on datasets that contain only small thoracic nodules, said Bram van Ginneken, PhD, professor of functional image analysis at Radboud University Medical Group in Nijmegen, the Netherlands, and Fraunhof Mevis in Bremen, Germany.
"This is a problem because they are much more suspicious for cancer," van Ginneken noted in a presentation at the 2014 RSNA meeting in Chicago. "When CAD is used as a second reader you may think this is not a problem because the radiologist sees the larger nodule. But if we move to CAD as a first reader, then this would be devastating."
Van Ginneken and colleagues Arnaud Arindra Adiyoso Setio, Jaap Gelderblom, and Colin Jacobs chose the publicly available Lung Image Database Consortium Research Project (LIDC/IDRI) database to analyze for the study, excluding scans with section thickness larger than 2.5 mm.
Included for analysis were all scans containing a solid nodule larger than 10 mm that were annotated by all four radiologist readers. (To be entered in this database the thoracic CT finding must be called one of four radiologist readers. In a second round, these readers were able to accept or reject marks proposed by other readers.)
Van Ginneken and colleagues ended up with 208 large nodules from 890 thoracic CT scans.
CAD chooses, refines candidates
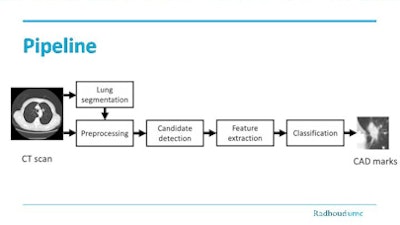
A problem with conventional lung segmentation may fail to include nodules attached to pleural wall, van Ginneken said. The investigators applied a special rolling ball algorithm (Sluimer et al) and dilation (Hu et al) to ensure the inclusion of these lesions.
In preprocessing regions outside the lung, they were masked to equal the dark "air" attenuation of the lung interior. Image resolution was standardized to resample to isotropic voxels of 1 mm3.
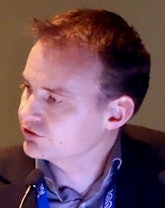
Large nodules have distinct morphological characteristics. Thresholding and open-based detectors should be sufficient for candidate detection, van Ginneken said.
"However, since they can be very large we often found that a single candidate detector is not optimal," he said. "So we have a candidate procedure that starts with intensity thresholding (-300 HU) but then we perform multiple morphological openings."
Once candidates are selected, a connected component analysis is applied to cluster them, nodule segmentation is refined (Kuhnigk et al), and nodule candidates too small (< 268 mm3) or too large ( > 33,524 mm3) are discarded.
Intensity features (mean, entropy, standard deviation) are then extracted inside the candidate segmentation and boundary box, and shape features (compactness, sphericity) are analyzed to exclude nonnondule candidates. Finally, a tenfold cross validation using a support vector machine (SVM) is applied.
High sensitivity, few false-positives
Sensitivity on conventional lung segmentation was 86%; applying the rolling ball improved it to more than 95%. Dilation also pushed sensitivity up, but additional lung border must be minimized, he said.
Overall, CAD detected 44.6 candidates per scan with a sensitivity of 205/208 large nodules, or 98.6%, van Ginneken reported.
After classification, "We got good sensitivity even at very low false-positive rate, and at high false-positive rates we detected almost all of the large nodules," he said.
False positives/scan | Sensitivity for nodules ≥ 10 mm |
1.0 | 85% (176/208) |
7.5 | 96% (199/208) |
"It is essential that CAD reaches high sensitivity in the detection of nodules because they are more likely to be cancer, and this special large nodule CAD can detect a large number of highly suspicious lesions with a small number of false positives," van Ginneken said. "You could merge the findings of this CAD system with the findings of other CAD systems and increase the total number of detected nodules."