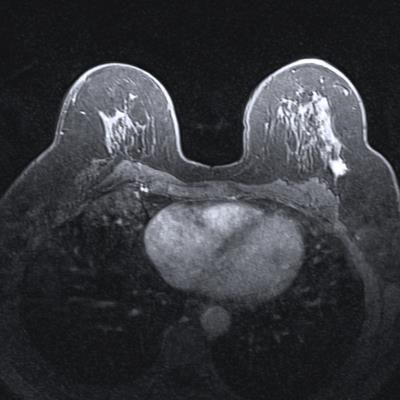
Artificial intelligence could help decrease radiologist workloads by nearly 16% when it comes to ultrafast breast MRI scans by identifying images most likely to have cancer, write Dutch researchers in a paper published on 26 May in European Radiology.
A team led by Dr. Xueping Jing from the University of Groningen in the Netherlands found that a deep-learning model they developed also showed high sensitivity and negative predictive values, as well as a nearly 17% scan time reduction.
"Reduced scanning and interpretation time could result in significantly lower breast MRI screening costs, making it possible to provide MRI screening for a wider population," Jing and colleagues wrote.
Dynamic contrast-enhanced MRI has advantages as a supplementary screening tool for breast cancer. These include detecting more breast cancer cases than mammography and detection at an earlier stage. Research also suggests that it can also reduce interval cancers, especially for women with extremely dense breasts.
However, cost-effectiveness is still a high barrier for wider use. Radiologists are looking at ways to improve this, including shortening image acquisition and interpretation times to reduce workload. Abbreviated protocols have been explored to reduce scanning times to less than five minutes.
Previous research suggests that AI can help by automatically excluding MRI scans without lesions. Jing et al wanted to develop and evaluate the feasibility of a deep-learning model that could automatically predict abnormalities.
In their retrospective study, the researchers looked at data from 837 breast MRI exams performed on 438 women from April 2016 to October 2019. The left and right breasts in each exam were labeled normal and without suspicious lesions, or abnormal and with suspicious lesions based on final interpretation.
Out of these, 178 exams from 149 women were included in an independent test set for the deep-learning model. Here, the women had an average age of 44 years.
The team found that the model achieved an area under the receiver operating curve of 0.81 for labeling exams as normal or abnormal. After applying a probability threshold of 0.25, the model had a sensitivity of 98%, a negative predictive value of 98%, a workload reduction of 15.7%, and a scan time reduction of 16.6%.
The researchers wrote that the challenge of using AI in triaging is that while a lower threshold for what constitutes a suspicious lesion is safer, it's less efficient. They added that the threshold is difficult to determine due to the trade-off between the risk of missing breast cancer and the reduction of workload.
The study authors called for double-blind, randomized clinical trials to further evaluate the model's performance, as well as the use of multiplanar maximum intensity projections to address masking effects.
Still, they wrote that an AI model could be a promising way to improve breast MRI screening's efficiency and accessibility.
"When integrated into the workflow, the model has the potential to reduce radiologists' workload by excluding normal scans and improving throughput by reducing scanning time," they wrote.