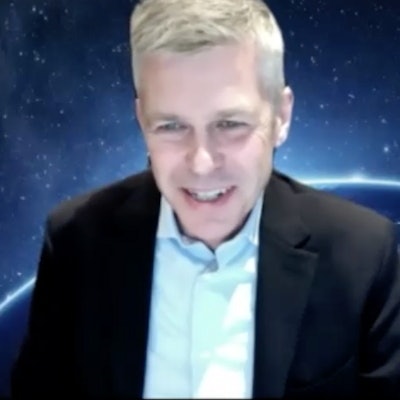
When it comes to artificial intelligence (AI), what lies ahead for clinical radiology? What are the main trends? These questions were addressed in a webinar on 12 January by an expert panel that included informatics expert Prof. Dr. Erik Ranschaert.
In this Q&A article, Ranschaert speaks about the obstacles to the clinical implementation of AI and the areas of special interest to him. He also makes some predictions about what may happen in 2022.
Ranschaert is a radiologist at St. Nikolaus Hospital, Eupen, Belgium, and visiting professor at Ghent University. He is immediate past president of the European Society of Medical Imaging Informatics, and has been an active member of the editorial advisory board of AuntMinnieEurope.com since the launch of the site at ECR 2011.
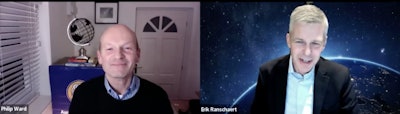
Q: What are the current barriers to implementing AI in radiology and what needs to be done to overcome these hurdles?
A: I think currently the five major barriers to AI adoption are the following:
- The limitations of performance and biases in AI applications. AI solutions need to be validated in a more stringent way when applied in a clinical environment and need to be monitored continuously to exclude the presence of any failure of the model.
- The current heterogeneous digital infrastructure. Ingestion of new solutions needs to be more streamlined; most hospital systems are still functioning separately. The infrastructure of most hospitals currently is not ready for seamless integration of AI-based solutions.
- The lack of technological literacy amongst its intended user base. There is no standardized curriculum for AI education nor are there relevant accreditation requirements within most Medical training programs.
- The ethical challenges involving multidisciplinary stakeholders.
- The financing issues: the lack of optimal business models to invest in AI solutions. A balance needs to be found between investing in quality/value vs. productivity.
On the last point, an interesting paper here is "Who will pay for AI?" by Melissa Chen and colleagues from the University of Texas (Radiology: Artificial Intelligence, 3 March 2021). The authors explain that as payment systems in healthcare progressively evolve towards more mature value-based payment models where measuring improvement in quality becomes increasingly important at decreased costs, AI is also likely to become a valuable and indispensable tool for radiologists and healthcare systems. The party who receives the most benefit from AI is likely to pay for it and ultimately may consider this payment simply the cost of doing business.
In many EU member states, the healthcare system and reimbursement on average incentivizes productivity to a larger extent than value/quality, at least from a financial perspective. We can only hypothesize that a significant shift towards value-based healthcare systems and, hence, quality-based reimbursement might also increase the perceived importance of quality and thus the value of using AI solutions.
Q: What specific radiology AI applications are you most excited about and why?
A: I think these four areas of particular interest right now:
- Algorithms providing support in analysis of complex examinations for clinical decision support, such as tools for analyzing prostate MRI scans and pulmonary CT scans, for both quantitative and qualitative analysis of findings (e.g., Contextflow and QP-Prostate)
- Algorithms providing feedback and quality control (e.g., Oxipit ChestEye Quality) -- quality is so important!
- Algorithms for automated analysis of ultrasound examinations of the thyroid gland or neonatal hips, for detection of congenital hip dysplasia, (e.g., Medo Thyroid)
- Algorithms for workflow monitoring (e.g. Equium Intelligence)
Q: What is the outlook for radiology AI over the short and medium term?
A: Although AI shows potential in improving the quality of life for patients by enhancing decision-making and tasks carried by healthcare professionals, the adoption of AI is rather incremental, and not causing a stark change in standard of care
We can see that AI is gradually being accepted as a new medical device, but the attention is gradually moving from excitement and fear for jobs to worries about the effect on patient care. At RSNA 2021, I noticed an increasing awareness about the necessity to obtain more evidence about the usefulness and value of AI applications, requiring thorough and continuous evaluation and monitoring of outcomes, even on a multicenter level.
Subject of debate are now topics such as the risks involved in the biasing of AI models, the assessment of the quality and accuracy of AI models in a real-life clinical environment, and the communication of results with clinicians and their acceptance or interpretation of AI results by clinicians. Another important issue is the seamless integration of results in workflow, including the automated integration of AI results and measurements in structured reports.
Q: What impact is the pandemic having on the evolution and implementation of AI? Has it markedly slowed the growth of AI?
A: A rather unintended consequence of the pandemic has been stimulation of the development and testing of AI diagnostic-aiding tools in radiology. I was involved in the development of such an algorithm for analysis of pulmonary CT scans in these patients. What we could see and experience is that many hospitals were suddenly willing to make their data available to create solutions that could be applied on a larger scale, especially from the perspective of the collective interest. Companies and medical professionals also wanted to spend time in creating such tools, largely on a voluntary basis. The pandemic also gave an impetus to cooperation between radiologists and software engineers, with the aim of jointly fighting this "invisible enemy" -- namely the virus.
Access to data is critical, but it has historically been monolithic, isolated, and difficult. Now hospitals, radiological societies, and even governments are starting to support image data collaboration. COVID-19 has brought a huge opportunity because significant R&D funds became available to facilitate image data sharing and collaboration. This is not only the case in the U.S., but also in Europe. For example, in the Netherlands, a national platform was created to collect radiological images for AI-related research purposes. This plan is called the Nederland COVID-19 Initiatief (NCIF).
Q: Can you gaze into your crystal ball and make some predictions about 2022? What hopes and expectations do you have for the year ahead?
A: More disruptive AI-based solutions are being developed and will become available, such as algorithms to create postcontrast CT images from native CT scans, eliminating the disadvantages and risks of intravenous contrast injections.
Most applications that are now available are related to image-based analysis and diagnosis. However, the low-hanging fruit applications of AI are the non-imaging based algorithms, such as upstream systems verifying radiological procedures and follow-up of findings, models to decrease motion artifacts and scanning times, tools to improve image quality, solutions to optimize capacity planning and to facilitate scheduling of examinations. I predict an increase in the development of such applications, since it's much easier to obtain the necessary certification, and the business cases are much easier to calculate. Nevertheless, for industry it seems to be a challenge to focus on this area.
There is a growing interest in AI applications for pathology, also for prostate and other tissues. We will see a boost of AI in the pharmaceutical industry, for genetic research and drug discovery, mainly in the field of neuroscience and oncology.
There will be growing activities among the big 3 (Google, Amazon, Microsoft) in healthcare. More computer power is becoming available, e.g., the new supercomputer of Microsoft Azure and the NVIDIA supercomputer in Cambridge. The acquisition of Nuance by Microsoft is also relevant to mention here. At RSNA 2021, the Nuance Precision Imaging Network was announced. This AI-powered cloud platform leverages best-in-class AI models to deliver data and insights from diagnostic imaging into existing clinical and administrative workflows.
More funding and grants for support of AI-based research will become available. Also, radiological societies will continue to adapt in terms of both education and research, as well as provision of new training opportunities for radiologists and others. For example, the RSNA is now organizing an online course to obtain a certificate in AI for radiology.
Editor's note: You can watch a recording of the AuntMinnieEurope.com and AuntMinnie.com webinar, "AI Trends in 2022: Clinical Radiology," on demand via this link. Further AI webinars will take place on 10 February and 6 April. The series is being produced by Brian Casey, editor in chief of AuntMinnie.com, and sponsored by Bayer.