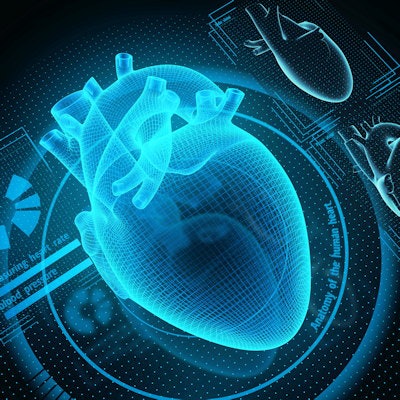
A research team in the U.K. has developed a novel deep-learning model capable of accurate, automated segmentation of high-resolution intravascular ultrasound images in real-time, according to a study published on 18 June in the International Journal of Cardiology.
A group led by Dr. Retesh Bajaj from Queen Mary University of London showed that the deep-learning model had high agreement with human readers.
"For the first time, we used a large number of high-resolution intravascular ultrasound images to train a deep-learning methodology that is based on the ResNet neural network and takes advantage of the adjacent intravascular ultrasound frames to accurately detect the external elastic membrane and lumen borders in frames," the authors wrote.
Preferred method
Intravascular ultrasound is the preferred method for assessing lumen and vessel wall dimensions, quantifying plaque burden, and guiding revascularization in complex lesions and high-risk heart patients. But its broader use is limited by the time and cost needed for acquiring and analyzing images. Bajaj's group called the process "laborious" and "time-consuming," and wrote that it relies on expert analysis.
Other methods have been introduced to help with automating images, but none have been shown to accurately identify the external elastic membrane and lumen borders in real-time while patients are present in the cardiac catheterization lab. So the investigators sought to develop and validate a deep-learning method capable of automated and accurate segmentation of intravascular ultrasound image sequences in real-time for larger datasets.
In the current study, two readers manually interpreted images for ultrasound segmentation. This included annotating the external elastic membrane and lumen borders in the end-diastolic frames of 197 intravascular ultrasound sequences that visualized the native coronary arteries of 65 patients. Intravascular ultrasound sequences of 177 randomly selected vessels were used to train and optimize the deep-learning model for image segmentation.
The team validated the model in 20 vessels, using the two readers' assessments as the reference standard. Average difference between the deep-learning model and the readers for identifying the external elastic membrane, lumen, and plaque area was at least 0.23 mm2; distance differences between the readers and the algorithm for the external elastic membrane and lumen borders were 0.19 mm or less.
Bajaj and colleagues also found that agreement between deep learning and the two readers was comparable to the readers' agreement, with similar results in frames portraying calcific plaques or side branches. The deep-learning algorithm was much quicker, however: Readers' manual segmentation of the end-diastolic frames took about nine hours, while the deep-learning model was able to segment vessels in two minutes.
"When analysis focused on challenging cases, such as frames portraying the origin of side branches or calcific plaques, we found that the deep-learning methodology performed well and that was as accurate as the analysts in assessing plaque area," the study authors wrote.
Overcoming artifacts
The researchers said that validation in such a large dataset has demonstrated that their deep-learning method is "able to overcome common artefacts seen in intravascular ultrasound and segment challenging frames portraying the origin of side branches and calcific plaques."
The team included frames showing common artifacts seen in intravascular ultrasound. These include guide-wire artifacts, nonuniform rotational distortion, motion artifacts, reverberations, side lobe artifacts, and blood speckle artifacts. Frames with calcified tissue and side branches were also included.
"These features are expected to facilitate its [deep-learning model] broad adoption and enhance the applications of intravascular ultrasound in clinical practice and research," the group wrote.
Future research projects will train the model to interpret lower-resolution images, according to Bajaj and colleagues: The current study's model iteration interpreted images at 50 MHz.
Finally, the group plans to expand the model's application to analyze stented coronary segments and incorporate automated detection and measurement of the arc of calcification, the authors wrote.