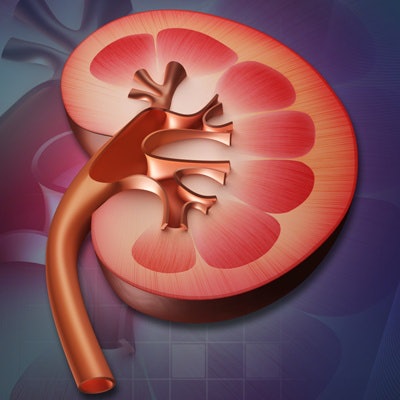
An artificial intelligence (AI) algorithm was able to identify kidney stones -- even very small stones -- at a very high level of accuracy on CT, according to research published online on 14 June in Computers in Biology and Medicine.
Researchers led by Dr. Kadir Yildirim of the University of Turgut Ozal in Malatya, Turkey, developed a deep-learning model to detect and localize kidney stones on noncontrast CT exams. The algorithm yielded 96.8% accuracy in testing and was deemed to be ready for clinical use.
"This study shows that recently popular [deep-learning] methods can be employed to address other challenging problems in urology," the authors wrote.
Seeking to apply AI to the challenge of preventing missed kidney stone diagnoses on CT, the researchers trained and tested a deep-learning model using CT images from 433 patients who had been admitted to Elazığ Fethi Sekin City Hospital in Turkey for suspected urinary system stone disease. These subjects included 278 with kidney stones and 165 without.
All images were acquired in the supine position on an Ingenuity Elite CT scanner (Philips Healthcare) without contrast. Using coronal CT images obtained from different cross-sections in these patients, the researchers produced a dataset of 1,799 images, which consisted of 790 images for patients with kidney stones and 1,009 of clinically normal subjects. A radiologist and a urologist separately evaluated the images and labeled them as either having kidney stones or no kidney stones.
Of the 1,799 images, 1,163 were used for training the algorithm and 290 were utilized for validation. The remaining 346 images were set aside for testing the model, an XResNet-50 cross-residual network.
The algorithm yielded the following performance on the test set:
- Sensitivity: 95.7%
- Specificity: 97.7%
- Accuracy: 96.8%
The authors noted that the algorithm performed well even on small kidney stones. In addition to identifying the presence of kidney stones, the algorithm also successfully marked the areas of interest it used during its decision-making process, according to the researchers.
"Clinically, the regions identified by the model [were] in agreement by our medical experts for most of the images," they wrote. "Hence, our proposed [deep-learning] model is accurate and can assist the radiologists to detect kidney stone cases accurately."
Yildirim and colleagues acknowledged the limitations of their work, including its reliance on images from only one hospital. They're currently planning to collect images from different sources and also intend to evaluate their method using axial and sagittal images. In addition, they said they would like to collect CT images with labeled kidney stone sizes to better understand the relationship between kidney stone size and algorithm classification performance.