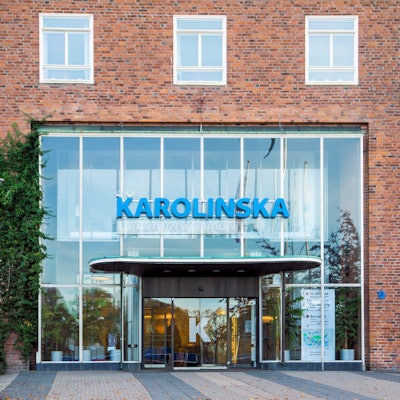
A breast cancer risk assessment model based on artificial intelligence (AI) outperformed traditional risk assessment tools in new research presented at the 2020 San Antonio Breast Cancer Symposium (SABCS) meeting. The AI model beat conventional risk models used alone and when combined with breast density data.
Researchers from the Karolinska Institute in Sweden created the algorithm using the institution's Karolinska Mammography Project for Risk Prediction of Breast Cancer (KARMA) cohort, which launched in 2011 and collects mammogram and blood-biobank data. This particular study focused on a subset of 974 women who developed incident cancer, as well as 9,376 healthy controls.
In the poster presented at SABCS 2020, researcher Dr. Mikael Eriksson, PhD, and colleagues showed that the KARMA model achieved an area under the curve (AUC) of 0.73. The AUC went up even further, to 0.74, when the team added the lifestyle factors and 0.77 when they added the genetic factors. The model also performed well in the two independent test sets, netting an AUC of 0.71 in a 9,849-person cohort and 0.73 in a 9,102-person cohort.
Women deemed high risk by the AI model ultimately had eight times the risk of developing an interval cancer compared with women in the general population. High-risk women were more likely to be diagnosed with stage II cancer and tumors at least 20 mm large, while they were less likely to be diagnosed with stage I cancer and estrogen receptor-positive tumors.
Performance of KARMA breast cancer risk assessment model | |
Model type | AUC (high to low) |
AI-based KARMA model based on mammogram image + genetic factors | 0.77 |
AI-based KARMA model based on mammogram image + lifestyle factors | 0.74 |
AI-based KARMA model based on mammogram image alone | 0.73 |
Polygenic risk score + mammographic density | 0.67 |
Polygenic risk score alone | 0.64 |
Tyrer-Cuzick + mammographic density | 0.62 |
Gail + mammographic density | 0.61 |
Tyrer-Cuzick alone | 0.58 |
Gail alone | 0.56 |
In addition, all three models outperformed conventional risk assessment tools, including the well-regarded Tyrer-Cuzick and Gail breast cancer risk models. The AI-based model also outperformed the traditional models both alone and when combined with breast density data.
The image-based algorithm parsed numerous mammogram features, such as density, masses, and microcalcifications, as well as left-right asymmetries and patient age. The researchers also extended the algorithm's capabilities using two different sets of factors:
- Lifestyle and familial factors, such as menopausal status, family history of breast cancer, body mass index, and use of hormone replacement therapy, tobacco, and alcohol
- Genetic factors, including a polygenic risk score with 313 polymorphisms
The research team developed the AI-based model as a tool to help reduce interval cancers. The goal is to better identify women likely to be diagnosed with breast cancer at or before their next screening mammogram.
"Mammography screening reduces breast cancer mortality, but a large proportion of
breast cancers are missed and detected at later stages or develop in between screening intervals," the authors wrote. "We developed the KARMA model which identifies women who are likely to be diagnosed with breast cancer before or at the next screen."