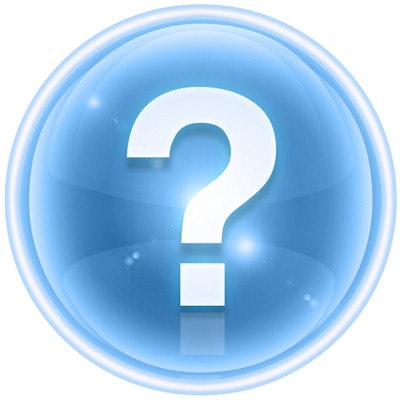
Artificial intelligence (AI)-based software can reliably categorize a significant percentage of negative screening mammograms as normal, potentially decreasing mammography reading workload for radiologists by more than half, according to two presentations made at the recent ECR 2020 virtual meeting.
In separate studies that simulated outcomes from the use of AI algorithms to evaluate screening mammograms and set aside certain cases that are highly likely to be normal, Danish and U.S. researchers shared their experiences about how software can decrease the interpretation burden of radiologists without having a negative impact on cancer detection.
Pressure on radiologists
With the vast amount of women enrolled in breast cancer screening programs worldwide, there's pressure on radiologists to read a substantial amount of mammograms -- the majority of which are normal. The use of AI to help radiologists automatically detect a large number of these normal mammograms could possibly increase the performance of the screening program, while also making it more effective, explained Andreas Lauritzen of the University of Copenhagen in Denmark.
In a retrospective study, the investigators assessed the potential clinical impact of using AI software to reduce the screening mammography workload, specifically examining cases where an AI system could substitute for two radiologists when mammograms are very likely to be normal, he noted.
The team analyzed 53,948 mammography exams acquired in the Danish Capital Region breast cancer screening program from November 1, 2012, to December 31, 2013, in women ages 50-70. All exams included four full-field digital mammography (FFDM) images -- two mediolateral oblique and two craniocaudal views -- that were acquired on a Mammomat Inspiration FFDM system (Siemens Healthineers). Two radiologists read each of the exams, with agreement established in consensus.
The 53,948 exams included 418 screening-detected cancers, 150 interval cancers, and 812 long-term cancers that were confirmed by mammography, ultrasound, and biopsy. There were also 1,306 exams that were noncancer recalls.
The researchers then retrospectively applied version 1.6 of the Transpara AI-based software (ScreenPoint Medical) to these exams. Transpara provides a score of 1-10 to indicate the likelihood of a visible malignancy.
The experiment was set up to have radiologists not read exams deemed by the software to be very likely normal and then double-read the rest of the exams. The researchers then compared the outcome of these experiments with the original screening outcomes.
Lower workload
Lauritzen and colleagues found that the AI software yielded an area under the curve (AUC) of 0.95 for screening-detected cancers and 0.66 for interval cancers. If an AI software scoring threshold of 5 was used, 32,054 exams would be considered likely normal and not read by radiologists, reducing mammography workload by 59.42%.
Screening mammography program outcomes in study of 53,948 screening mammograms from Danish Capital Region | ||
Original screening outcomes | Screening outcomes if AI software scoring threshold of 5 was automatically used to determine a normal mammogram | |
Recall rate | 3.18% | 2.48% |
Positive predictive value | 24.34% | 30.04% |
With this strategy, 16 (3.83%) of the screening-detected cancers that were detected during the normal double reading process would have been missed. However, if this AI strategy was expanded to recall all women with an exam score > 9.96, 16 new cancers would be detected, including five interval cancers and 11 long-term cancers. These added detections would come at the cost of only 91 new noncancer recalls, Lauritzen said.
This demonstrates that it's possible to maintain a stable cancer detection rate and still avoid a large number of noncancer recalls, he pointed out.
"This study suggests that an AI system can be used to maintain safety of the breast screening program, possibly increase performance, while reducing the number of mammograms that have to be read by radiologists by a considerable amount," Lauritzen said.
More study needed
However, this recall threshold would still need to be studied in detail before being placed into clinical use, Lauritzen said.
He noted that the study considered an extreme scenario of replacing both radiologists for certain mammograms.
"The more likely scenario is to replace a single radiologist," he said. "However, this study remains clinically relevant as it demonstrates the clearly positive impact [of the software] on a screening program.
Also, "a limitation of this study is that the radiologists would only read exams with high AI scores," he said. "Being aware of that fact might affect their performance and this effect will be studied in a future prospective study."
Improving reader performance
In a second study, Dr. Alyssa Watanabe, chief medical officer of CureMetrix in Manhattan Beach, California, reported that the company's cmTriage deep learning-based software could also yield significant workflow reduction for radiologists and improve their performance.
After assessing the performance of the AI software on two different datasets, the researchers found that it could potentially yield workflow reductions as high as 62% for radiologists. It could also help avoid missed cancers, according to Watanabe.
"This research shows that cmTriage can potentially be used for workflow reduction, either as a standalone or through elimination of double reading, while at the same time improving reader performance," she said.
The researchers assessed the performance of cmTriage on two test sets, including an enriched set of 1,255 screening 2D digital mammograms from three different imaging facilities and multiple imaging vendors. This test set included 400 biopsy-proven cancers and 855 negative cases.
Operating on a 99% sensitivity setting, the cmTriage software could have reduced radiologist workflow by 40% in this enriched test set without missing any cancers, according to Watanabe.
They also evaluated the software on a batch of 1,129 cases of 2D screening mammograms, representing three months of data from a single academic breast center. No cases were excluded, and the cancer prevalence rate was 7 per 1,000.
Screening outcomes on three full months of data from an academic screening center | ||
Without AI triage software | With AI triage software at 93% sensitivity setting | |
Number of cases categorized as very low suspicion of cancer by software | n/a | 1,138 (62%) of 2,129 screening mammograms. |
Recall rate | 13.4% | 7.4% |
Biopsies | 61 (15 cancers) | 53 biopsies (15 cancers) |
All of the cases categorized by the software as very low suspicion of cancer were confirmed as negative during follow-up of more than one year. In addition, all of the cases with cancer were correctly sorted as suspicious by the software. There were three missed cancer cases, all of which were flagged correctly by the software, Watanabe said.
"So in this real-life scenario, there was potential for not only workload reduction but over 50% recall reduction and a potential for 12% biopsy reduction," she said. "And in addition, there were the several missed cancers and the potential to increase the cancer detection rate by 17%, which would be an additional one per 1,000."