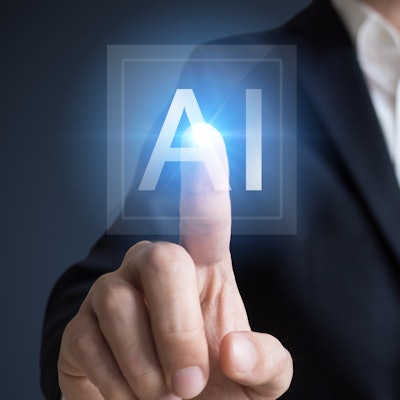
Making use of radiomics, an artificial intelligence (AI) algorithm can accurately differentiate between benign and malignant thyroid nodules on ultrasound exams, perhaps even better than some radiologists can, according to a study published online on 12 April in the European Journal of Radiology.
A team of researchers led by Hui Zhou, PhD, of the University of Chinese Academy of Sciences developed deep-learning radiomics of thyroid (DLRT), an algorithm that can automatically perform quantitative analysis of ultrasound images to characterize thyroid nodules. In testing on an external validation test set, DLRT achieved an area under the curve (AUC) of 0.97, outperforming other deep-learning algorithms as well as two ultrasound radiologists in the study.
"It holds great promise for improving the differential diagnosis of benign and malignant thyroid nodules," the authors wrote.
Subjective modality
Although most thyroid nodules on ultrasound are clinically significant, approximately 10% of patients with these nodules are at risk of cancer, according to the researchers. As a result, it's vital to accurately identify benign and malignant thyroid nodules for appropriate clinical decision-making and patient management. Ultrasound remains a highly operator-dependent and subjective modality for diagnosing thyroid cancer, however.
To see if a radiomics approach could more accurately distinguish between malignant and benign nodules, the researchers created DLRT, a convolutional neural network (CNN)-based transfer learning method for quantitative analysis of thyroid ultrasound images.
The algorithm was trained and validated using ultrasound images and fine-needle aspiration biopsies from 1,629 patients from the Ningbo No. 2 hospital in China, including 1,003 benign nodules and 642 malignant nodules. Of these 1,629 cases, 1,097 were used as the training cohort and 532 were set aside as an internal validation set.
They then compared the performance of DLRT with that of a basic CNN model, a transfer learning model, and two ultrasound radiologists (one with 12 years of experience in thyroid diagnosis and one with three years) on an external validation test set of 105 thyroid nodules from a different institution: HwaMei Hospital. These cases included 75 benign nodules and 30 malignant nodules.
As all ultrasound images in the study were acquired on scanners from two different vendors (Esaote and Philips Healthcare), the researchers also assessed DLRT's performance across different systems.
Performance for distinguishing between benign and malignant thyroid nodules on external validation test set | |||||
Basic CNN | Transfer learning model | Radiologist 1 (senior radiologist) | Radiologist 2 (junior radiologist) | DLRT model | |
Area under the curve | 0.82 | 0.87 | N/A | N/A | 0.97 |
Sensitivity | 65.1% | 78.3% | 64.2% | 65% | 89.5% |
Specificity | 88.2% | 81.2% | 75.5% | 62.5% | 84.1% |
Positive predictive value | 78% | 80% | 78.1% | 75.1% | 87.5% |
Negative predictive value | 79.1% | 81.1% | 64.2% | 62.3% | 87.5% |
The AUC of the DLRT model was significantly higher than the AUCs for the other two deep-learning algorithms included in the study (p < 0.01). Its higher sensitivity and specificity than the human observers was also statistically significant (p < 0.001). Notably, the algorithm performed at almost the same AUC on both types of ultrasound equipment in the study.
"After observing its analytical pattern on transferred heat maps, we recognized that the nodule surrounding adjacent parenchyma was vital for classification, especially for these challenging cases in human eyes," the authors wrote. "This deep learning visualization technique is likely to assist radiologists for more efficient interpretation of thyroid [ultrasound] images."
Further validation needed
The researchers noted that their approach now needs to be further validated in a multicenter prospective study.
"A larger dataset acquired from different hospitals with more types of [ultrasound] instruments is necessary for consisting a more comprehensive training cohort, so that the accuracy and reliability of DLRT can be continuously improved for each [ultrasound] scanner, as well as the question of whether it will have worse performance on certain [ultrasound] scanners can be properly addressed," they noted.