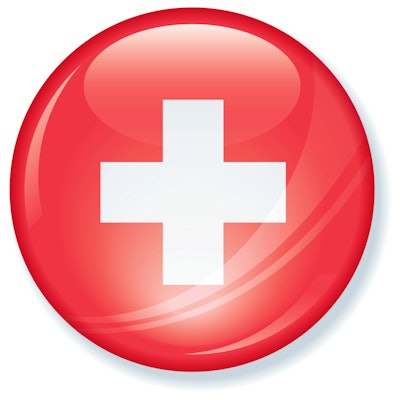
An artificial intelligence (AI) algorithm can accurately reconstruct 4D flow MRI exams in seconds, potentially enabling near-real-time assessment of cardiac blood flow while the patient is still in the scanner, according to research published on 13 April in Nature Machine Intelligence.
Using a small training dataset, researchers led by Dr. Valery Vishnevskiy of ETH Zürich in Switzerland developed FlowVN, a deep‑learning model that can reconstruct cardiovascular blood flow in 4D as accurately as conventional reconstruction methods, but in a fraction of the time on standard computers.
"Remarkably, the relatively low amounts of tunable parameters allowed the network to be trained on images from 11 reference scans while generalizing well to retrospective and prospective undersampled data for various acceleration factors and anatomies," the authors wrote.
Although 4D MRI can be utilized to reconstruct 3D cardiovascular blood flow over time and are important for diagnosing a range of cardiovascular diseases, the modality typically requires up to 20 minutes of image processing time. That means these exams will usually be assessed after the patient has left, according to the researchers.
"Speeding up these scans would allow real-time assessment while the patient is still in the scanner, potentially freeing up a clinician's time and decreasing patient discomfort," according to a press release from Nature.
Using a limited training set of only 11 studies, the researchers trained FlowVN, a deep variable neural network that replicates the 10 steps of an iterative reconstruction technique, but with several added modifications that speed up performance while maintaining accuracy. They then compared the reconstruction results of FlowVN with that of a traditional compressed-sensing iterative reconstruction method, as well as a 3D deep-learning model called HamVN that was adapted for 4D.
In both retrospective and prospective evaluations of seven healthy volunteers and one patient with abnormal blood flow, FlowVN was able to reconstruct aortic flow as accurately as the conventional techniques and better than the other deep-learning model, according to the researchers. It was also much faster despite being more complex than the other models.
Typical reconstruction times and model complexity for 4D flow reconstruction | |||
Compressed-sensing locally low-rank reconstruction (CS-LLR) | HamVN deep-learning model | FlowVN deep-learning model | |
Reconstruction time | 10 minutes, 24 seconds | 89 seconds | 21 seconds |
Number of parameters | 2 | 62,742 | 63,583 |
"The proposed reconstruction is 30 times faster than state-of-the-art CS-LLR and 4.2 times faster than HamVN due to the use of linear activation functions rather than radial basis functions, which require computation of pairwise distances between control knots and image intensities," the authors wrote. "It is worth noting that FlowVN demonstrates high generalization ability, being able to preserve patient pathologies that were not present in the training data."
The researchers credited their modifications to the FlowVN network architecture with producing a model that can better adapt to data, yield higher accuracy, and provide better generalizability.