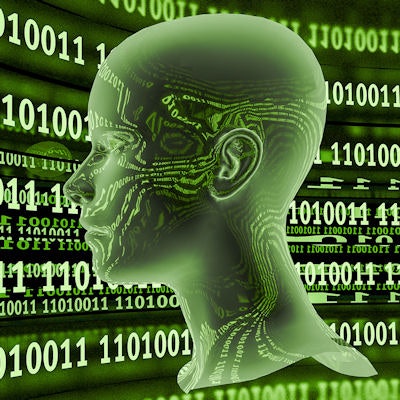
Different CT tube voltage levels didn't hinder the performance of a prototype artificial intelligence (AI) algorithm for estimating fractional flow reserve on coronary CT angiography (FFR-CT), according to a study by a multinational research group published on 30 April in the American Journal of Roentgenology.
The researchers led by Dr. Jakob De Geer, PhD, of the Center for Medical Image Science and Visualization in Linköping, Sweden, retrospectively assessed a prototype commercial machine-learning algorithm on more than 300 coronary CT angiography (CCTA) scans at different voltage levels. They found no statistically significant differences in the algorithm's performance.
"Hence, [the machine-learning algorithm] seems to be a robust technique with comparable performance," the authors wrote.
Although CCTA-based methods can noninvasively estimate FFR, it's unknown whether the varying attenuation values produced by different tube voltages significantly affect the diagnostic performance of these techniques, according to the researchers. As a result, the team sought to retrospectively evaluate the effect of tube voltage on the performance of a machine-learning algorithm trained to estimate FFR.
In their study, the researchers utilized data from the Machine Learning-Based CT Angiography-Derived FFR: A Multi-Center Registry (MACHINE) consortium -- a collaboration among five institutions in North America, Europe, and Asia to evaluate machine-learning applications for FFR-CT. Their database included a total of 351 patients and 525 vessels; 174 patients (265 vessels) had been scanned with a tube voltage of 100 kVp, 160 patients (225 vessels) had been imaged with a tube voltage of 120 kVp, while 17 patients (35 vessels) had been examined with a tube voltage of 80 kVp.
All patients received their CCTA study on one of two models of commercially available dual-source CT scanners (Somatom Definition or Somatom Definition Flash, Siemens Healthineers). Images were reconstructed using a medium-smooth kernel and a slice thickness of 0.75 mm or less with an increment of 0.4 mm. These exams were then processed by version 2.1 of a machine learning-based software prototype, called cFFR, currently being developed by Siemens. The researchers then compared the algorithm's performance with FFR results acquired via invasive coronary angiography.
Impact of tube voltage on FFR-CT machine-learning algorithm | ||
100 kVp | 120 kVp | |
Spearman correlation coefficient | 0.622 | 0.669 |
Intraclass correlation coefficient | 0.76 | 0.77 |
Sensitivity | 73.5% | 85% |
Specificity | 79% | 72.8% |
Area under the curve | 0.82 | 0.84 |
The differences weren't statistically significant. In other findings, the researchers observed that the 17 patients who were imaged with a tube voltage of 80 kVp seemed to show even better correlation and agreement with invasively measured FFR. They weren't able to conclusively determine the reasons why, however, due to the small sample.
The authors also acknowledged that although the first- and second-generation dual-source CT scanners used in their study still meet the current recommendations for CCTA, state-of-the-art scanners can scan the coronary arteries with even lower tube voltage, less contrast medium, and lower dose.
"Thus, further research in this area is warranted, especially as the possibilities of using advanced postprocessing as a complement to visual evaluation are ever increasing," the authors wrote.