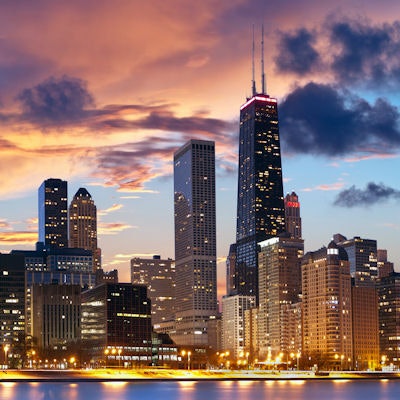
An artificial intelligence (AI) algorithm can accurately detect vertebral fractures on CT images containing the spine, enabling opportunistic screening of these patients, according to research presented on Sunday at the 2019 RSNA meeting.
In a feasibility study, a team of Dutch researchers trained a 3D convolutional neural network (CNN) to localize and grade vertebral fractures in a manner that's consistent with clinical practice, according to presenter Joeri Nicolaes, a computer scientist at pharmaceutical firm UCB Pharma. In testing, their model achieved an area under the curve (AUC) of 0.93.
"We believe that it's really important to try and identify patients who [have not yet been diagnosed with severe osteoporosis] by actually opportunistically screening for vertebral fractures in CT images of patients older than 55," Nicolaes said. "We are truly committed to try and tackle this unmet need and make sure that these patients don't have to suffer a second [osteoporotic] fracture before they get into the clinical system."
Every three seconds a fragility fracture occurs somewhere in the world, and spinal fractures are some of the most common, according to Nicolaes. These fractures are often asymptomatic and go unnoticed, but they are important risk-predictors for early signs of osteoporosis. However, 54% of vertebral fractures are underreported by radiologists in spine-containing CT exams, Nicolaes noted.
"These two findings motivated us to work on ... approaches to screen for vertebral fractures in CT and trying to reveal those for prevention and avoidance of secondary fractures," he said.
Radiologists currently assess for vertebral compression fractures using Genant's semiquantitative technique. A number of semiautomated methods can also mimic the human approach and work in two stages: segmentation of the vertebrae and then detection of vertebral compression fractures based on 2D or 3D computed features, according to Nicolaes.
Recently, deep-learning methods have also been applied to automatically map an input CT image and process 2D sagittal slices to provide a binary output of whether or not a patient has a fracture, he said.
In their project, Nicolaes and colleagues used a 3D CNN to automatically learn compact 3D features for detecting vertebral compression fractures. It doesn't require prior segmentation of the spine or selection of the appropriate sagittal slice.
They trained the model on 90 CT images that were acquired on three different types of scanners for various indications. The dataset was curated by one reader who scored the Genant grades for every vertebra present in the field-of-view. This dataset contains 969 vertebrae, 19% of which were vertebral compression fractures.
After performing fivefold cross-validation, the researchers found that their algorithm produced an AUC of 0.93.
The project was an initial feasibility study, and the researchers now want to integrate a vertebra localization method into the pipeline to provide fully automated vertebra-level fracture detection. In addition, they would like to be able to predict Genant fracture grades.
"This will eventually lead to clinically relevant results," Nicolaes said.
In addition, the researchers are working to extend the training dataset to include more thoracic vertebrae and other spine pathologies. Finally, they plan to verify the robustness of their method in external validation experiments, he concluded.