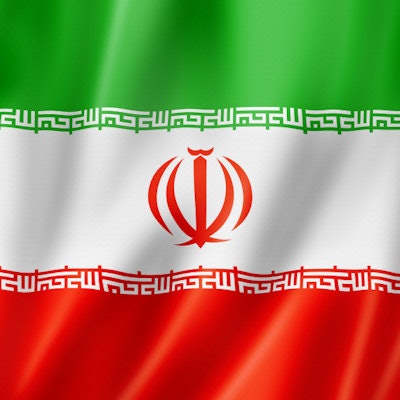
Researchers from Iran used a machine-learning algorithm and perfusion-weighted MRI scans to differentiate between patients with uterine sarcomas and those with leiomyomas. The findings were published on 12 November in the European Journal of Radiology.
Although uterine sarcomas are rare -- accounting for only 3% to 9% of uterine cancers -- distinguishing between sarcomas and fibroids can be tricky, and surgery has long been the primary diagnostic tool, wrote the group led by Dr. Mahrooz Malek from Tehran University of Medical Sciences.
"Currently there [are] no reliable diagnostic criteria for differentiating uterine sarcomas from other uterine masses and usually the ultimate diagnosis can be made only after surgery based on postoperative histopathological assessment," the researchers wrote.
Malek and colleagues investigated whether sarcoma and fibroid characteristics extracted from perfusion-weighted MRI exams could be incorporated into a machine-learning algorithm that would help clinicians better distinguish between the two. The study included 42 women with a total of 60 masses, 10 of which were cancer and 50 of which were fibroids. All of the women underwent a standard MRI exam to assess these masses, followed by another exam using the perfusion-weighted MRI technique.
Radiologist readers flagged two regions of interest (ROIs) on the images: the entire tumor and the area of the lesion with the most contrast enhancement. Next, two additional areas of interest were marked on the psoas muscle and the myometrium for baseline comparisons. Data from these exams were then used to train the algorithm, DCE Tool, which is available within the ClearCanvas image storage and visualization framework.
DCE Tool gives users the ability to model contrast uptake by considering blood plasma and the extracellular extravascular space as two compartments, and it computes metrics for kinetic parameters that govern uptake. DCE Tool also provides four semiquantitative parameters for characterizing the analyzed ROIs, according to the authors.
When the researchers fed data from the marked areas of interest for the entire tumor and the lesion with the most contrast enhancement into the classifier, its overall accuracy for distinguishing sarcomas from leiomyomas was 66.7%. But when they added the baseline data from the psoas muscle and the myometrium, the algorithm's performance improved to an accuracy rate of 91.7%, a sensitivity rate of 100%, and a specificity rate of 90%, Malek and colleagues found.
The study results show that machine learning can effectively help clinicians discern between benign and malignant masses, the group concluded.
"These preliminary results suggested that the proposed method could be potentially utilized along with conventional MRI sequences to differentiate between sarcomas and leiomyomas," the researchers wrote.