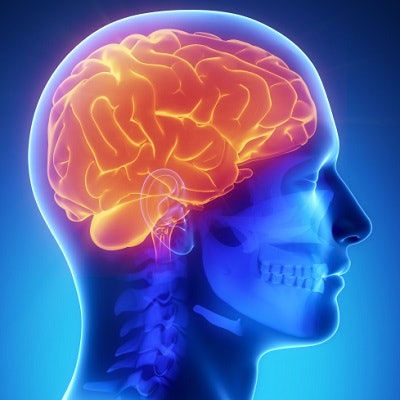
Researchers from the U.K. have developed an artificial intelligence (AI) algorithm capable of accurately detecting and rating the severity of brain lesions on CT scans of patients presenting with stroke or dementia, according to an article published on 15 May in Radiology.
The group's machine-learning algorithm was able to identify white-matter lesions and score the severity of small-vessel disease just as well as a team of neuroradiologists and stroke physicians on a dataset of more than 1,000 CT scans of stroke patients from 70 hospitals throughout the U.K. (Radiology, 15 May 2018).
With a rapid processing time and an 85% correlation to MRI, the current gold standard technique for diagnosing small-vessel disease, using the algorithm to evaluate patients presenting with stroke or dementia could lead to better treatment and care in everyday practice, senior author Dr. Paul Bentley, PhD, from Imperial College London said in a statement released by the university.
"Current methods to diagnose the disease through CT or MRI scans can be effective, but it can be difficult for doctors to diagnose the severity of the disease by the human eye," he said. "The importance of our new method is that it allows for precise and automated measurement of the disease. This also has applications for widespread diagnosis and monitoring of dementia, as well as for emergency decision-making in stroke."
Automated assessment
Cerebral small-vessel disease, or leukoaraiosis, is a common neurological condition that reduces blood flow to the brain and can lead to stroke or dementia. Clinicians most frequently identify the disease by detecting changes to white-matter lesions and lacunar infarcts on fluid-attenuated inversion-recovery (FLAIR) MRI or CT scans of the brain.
Though FLAIR MRI offers the highest sensitivity for these brain lesions, CT has long been the more commonly used modality for the targeted population, which includes patients presenting with stroke, traumatic brain injury, or dementia, according to the authors.
However, characterizing the severity of white-matter lesions on CT often leads to imprecise and variable results due to the limited visibility of lesions on CT compared with MRI scans, as well as the subjective nature of grading lesions using visual criteria.
To improve the assessment of white-matter lesions on CT, Bentley and colleagues developed a machine-learning algorithm capable of automatically segmenting them on CT scans and subsequently determining their volume.
The researchers first trained the algorithm using a set of 120 images. Next, they tested its accuracy on three different cohorts of patients examined at various U.K. hospitals between 2000 and 2014: 627 patients who experienced acute ischemic stroke and received thrombolysis treatment, 255 acute ischemic stroke patients who underwent CT and MRI within one week of each other, and 200 participants in the Third International Stroke Trial (IST-3).
Three among a pool of 13 experts -- neuroradiologists or stroke physicians with training in tracing and rating brain lesions -- segmented and rated the MRI and CT scans for each case. The researchers then calculated the total lesion volume from these tracings and compared the volumes with those collected with the automated machine-learning algorithm.
Comparable accuracy
In the 60 cases that included both CT and MRI data, the algorithm's estimates of white-matter lesion volume had a high correlation with the volume estimates made by experts on FLAIR MRI scans, even after adjusting for age, sex, and common CT features such as a previous infarct. At 85%, the algorithm's accuracy topped the accuracy of expert readings on CT scans by more than 3% and was approximately 8% shy of matching the accuracy of expert readings on FLAIR MRI scans.
Manual tracing vs. algorithm for white-matter lesions on MRI and CT | |||
Expert manual tracing | CT algorithm | ||
MRI | CT | ||
Accuracy | 93.7% | 81.9% | 85% |
Median tracing time | 7.9 minutes | 109 seconds |
It took the experts more than four times longer to complete their manual tracings than it took the algorithm for both image preprocessing and tracing. In addition, rating the lesions' degree of severity took the experts approximately 1.5 minutes to 2 minutes per image, compared with several seconds for the algorithm.
Despite their high accuracy, the automated lesion volume measurements were smaller than the expert readers' lowest volume measurement in 43% of the cases (p < 0.001). The algorithm also produced a slightly higher number of false negatives but slightly fewer false positives than the expert readers did.
What's more, the level of agreement between the algorithm and the experts as a whole (0.599) was greater than the interobserver agreement among individual experts (0.506) for rating lesion severity (p < 0.001).
The highly accurate and rapid reading of white-matter lesions by the algorithm demonstrates its potential to assist clinicians in the treatment of and decision-making process for patients with emergent neurological conditions, Bentley said. For example, the algorithm's capacity to evaluate white-matter lesions may help determine a patient's risk of hemorrhage, which, in turn, could inform physicians on whether they should administer clot-busting medication.
'First step'
Beyond stroke care, the machine-learning algorithm may also be useful for cases of dementia by quantifying the likelihood that patients will develop the condition as a consequence of small-vessel disease, Bentley noted. Furthermore, developments to the algorithm may allow for more personalized medical care.
"This is a first step in making a scan reading tool that could be useful in mining large routine scan datasets and, after more testing, might aid patient assessment at hospital admission," said Dr. Joanna Wardlaw, co-author and head of neuroimaging sciences at the University of Edinburgh.
The investigators are now using machine-learning algorithms to measure brain mass and shrinkage, as well as aid the diagnosis of other types of brain conditions frequently diagnosed on CT.