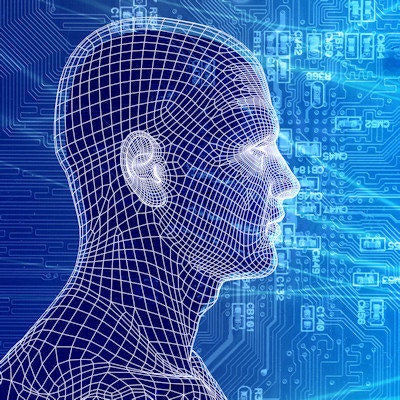
The major contribution of artificial intelligence (AI) to medicine comes from the automation of routine functions, which can result in considerable time savings. Hence, the major driver for the further development of AI is the economics of reducing costs.
According to an estimate from McKinsey, the management consulting firm, automation of healthcare can result in a saving of approximately 7%. This also explains why automation is mostly cherished by corporate, regional and national healthcare systems, insurance companies, large hospital networks, and contract research organizations.
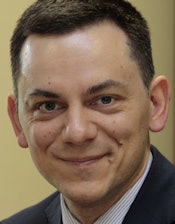
One-third of healthcare processes can be automated, estimates CB Insights. The most vulnerable functions are the routine and "mechanical" ones, such as data collection and data analysis. For example, the work of technologists/radiographers is gradually becoming more standardized, and thus at higher risk for automation, with an estimated potential of more than 60%. Radiology in particular is prone to automation, with a reported potential of 30% to 60% to substitute human functions by computer algorithms.
Already many imaging systems are available with a highly automated scanning process, which allow for a significantly reduced scanning time of the patient. Automated ultrasound scanners (e.g., automated breast ultrasound [ABUS] from GE Healthcare) and compact handheld scanners (e.g., Lumify from Philips Healthcare) contribute to this significantly. Furthermore, AI software is being developed to automate the reading of the images created by such scanners, so that even nonradiologists or nonmedical doctors could perform these examinations.
The following major trends and ongoing shifts in radiology are providing a basis for further automation:
- From analogue to digital data
- From modality (CT, MRI, x-ray, etc.) to pathology-oriented specialization
- From "jack-of-all-trades" roles (a physician or technician performing multiple functions) to delegation of functions (e.g., from radiologist to registrar, technician, nurse, clinical physicist, IT-specialist)
- From separated to integrated clinical facilities
- From art to craft and skills
- From unique services to mass production (commoditization)
The value and quality of radiology, and thus the strengths, are defined by the following domains:
- Appropriateness -- in a timely and efficient way
- Diagnostic accuracy -- technical and clinical
- Safety -- physical, mental, informational
- Actionable reporting -- standardized, structured, clinically valuable
- Communication -- proper and timely
The proper way of defining the ideal role of AI in healthcare and radiology is by first identifying the existing sources of waste and inefficiency, and by consecutively finding an appropriate solution to eliminate them. The problems and sources of waste in radiology include the following:
- Inadequate quality of studies -- around 15% to 20% with diagnostic discrepancies
- Shortage of high-quality (sub)specialists
- Insufficient communication with patients and referring physicians
- Lack of confidentiality, vulnerability of data
- Low efficiency, mostly represented by time inefficiencies
The major reasons for time-wasting in radiology are the following:
- Selection of imaging study and writing of referral by referring physician
- Scheduling a study
- Waiting time for a study
- Completing questionnaires
- Waiting for a study to begin
- Selection of appropriate study type by technician/physician
- Technician optimizing an appropriate scanning protocol
- Radiologist skimming through all images, including normal ones
- Comparison with priors without presaved key-images
- Reconstruction of images, postprocessing
- Drafting of report
- Corrections and explanations of reports
The existing (real) and possible future (potential) applications of AI in radiology are the following:
- Data collection - real application
- Questionnaires with electronic health record (EHR)-integration and automated data retrieval
- Referrals with automated assignment of modality and scanning protocol for a particular diseases and ICD codes ("clinical decision support" system)
- Visibility and accessibility of scheduling
- Generation of an extract of relevant clinical data and history
- Informed consent with an electronic signature
- Scanning with standardized and predefined protocols
- Automated retrieval of priors, automated comparison
- Data processing - real application
- Analysis of questionnaires
- Highlighting abnormalities on images
- Image reconstruction
- Automated comparison with priors
- Report generation, interactive-type of structured reports
- Peer-review
- Managing other people -- potential application -- based on multiple soft skills and direct communications
- Applying expertise -- potential application -- based on consultations and clinical integration
- Interactions with referring physicians and patients -- potential application -- based on communication skills and methods
- Unpredictable physical work -- potential application -- based on personal interaction, such as nursing care
AI algorithms are the most promising for automation of routine, well-described workflow functions, such as the following:
-
Triage and preparation of relevant images prior to reporting
- Automated identification of studies that are most probably normal, which requires a high sensitivity of the AI algorithm
- Automated identification of studies with pathologic findings for further analysis and reporting, which requires a high specificity of the AI algorithm
- For instance, background analysis of joint x-rays for skeletal age, of chest low-dose CT for lung cancer screening, of plain abdominal CT for renal colic, of CT angiography for pulmonary embolism, etc.
-
Standardized measurements
- Automated delineation and measuring of lesions and pathologic findings in standardized workflows
- For example, Response Evaluation Criteria in Solid Tumors (RECIST) assessment and follow-up of oncologic studies.
-
Semiautomated reporting
- Standardized templates
- Automated scoring, e.g. PI-RADS, BI-RADS, LI-RADS, Lung-RADS, Rectal-MR-RADS, Colon-CT C-RADS
- Automated coding of reports for research and data mining
- Translation and style adaption of reports according to reader's profiles (referring specialist versus general practitioner versus patient)
-
Quality assurance
- Radiologist's report analysis for inconsistencies, checking for a completeness of a study, checking for artifacts. All of these can be expressed by relevant metrics.
With the development of new technologies such as AI, it frequently happens that new applications are being introduced that don't fit in the healthcare workflow. It usually happens when the idea for a new tool comes from a task that is locally important but not scalable. The best way to develop applications is to start with identifying a problem hampering the workflow on a larger scale, and from there to develop a relevant AI-based solution for that problem. The biggest problems are the biggest business opportunities.
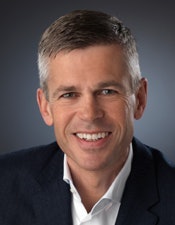
One such problem is a worldwide shortage of experts in radiology who are able to provide 95% to 100% accuracy in making a diagnosis. This is obviously a driver for booming teleradiology services around the world. In the next few years the value and usage of teleradiology can be strengthened by adding services such as automated structured reporting, including language processing for translational purposes, and automated triage of studies for outsourcing.
The use of blockchain services (BaaS, blockchain as a service) could allow safe cloud-storage of patient data and thus significantly decrease the cost of data transmission for telemedicine and teleradiology purposes. All this will contribute to the creation of a new expert-as-a-service (EaaS) working model for radiologists and other specialists, in which it will be possible to offer their services in a much wider area. The discussions on the usage of such EaaS model will be difficult, especially regarding the legal requirements for practicing medicine elsewhere, but the model will tremendously increase the availability of experts on a global scale.
What's next?
According to Jeremy Howard, famous data scientist and founder of the company Enlitic, "AI is going to be simple to use." However, most machine-learning protocols are thrown away as they are not based on future business models and standardized workflows. According to Neil Jacobstein, who chairs the Artificial Intelligence and Robotics Track at Singularity University, a think tank in Silicon Valley, California, "AI comes with certain trade-offs, such as jobs disruption, and even change of human identity." He also thinks these risks are relevant to all job levels. As data scientists and physicists are leading the development of new AI algorithms for healthcare, their roles might be automated in the future as well.
The following incentives for developing more AI tools in healthcare are very strong, and mostly related to economics and efficiency:
- Optimization of payroll by eliminating low value functions and increasing efficiency
- Increased quality be reducing mistakes due to wrong identification of patient or protocol
- Optimization of costs related to clinical trials by standardizing image assessment in trials with multiple interval imaging studies
- Controlling costs by eliminating inappropriate studies
The widespread introduction of AI algorithms and services into the clinical workflow is currently limited by a number of factors, the major one being the lack of clinically tested and confirmed products. Additionally, the absence of national legislations regarding the usage of patient data for AI algorithms limits the wider and faster development of algorithms. Many governments are disinclined to allow sharing of patient data, which is necessary for training algorithms (big data). Currently, however, the further development of AI is being fostered and streamlined by several countries such as China, Canada, and Brazil, which are in the lead with strategically adopting AI in healthcare.
New Europlean regulation
The European General Data Protection Regulation (GDPR), which will be effective starting May 2018, clearly defines and allows the usage of clinical data for sharing and research applications. The legislation will facilitate the development of clinically justified AI algorithms on the European level. Hence, starting from 2019 we shall see a bounty of scientific presentations on the clinical use of AI algorithms.
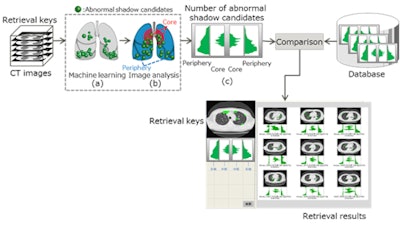
AI will also leverage the field of radiomics by helping to find connections between different types of data coming from clinical, pathology, radiology, lab, and genetics databases (radiogenomics). Integration of personal monitoring data with the EHR will allow the correlation of currently isolated metrics, such as an individual's number of footsteps, coronary calcium score, or visceral fat percentage as measured by CT, with personal health data and gene patterns. This will open a new era of high-precision prediction models and of "personalised medicine," which will improve the monitoring and management of many diseases on a personal patient-related basis instead of a more population-based decision model.
AI is a part of an entire technological ecosystem, including cloud storage and computing, blockchain and telemonitoring devices. Blockchain technology is able to permanently track all transaction results in any industry. Blockchain-based medical record systems could improve interoperability between healthcare providers while preserving the patient's' ownership of their personal data.
If we look at AI as the motor of an electric car, then cloud storage would represent the batteries, blockchain the electronic framework providing a reliable and sustainable interoperability of all car components, the imaging modalities and telemonitoring devices would be similar to the car's sensors and cameras, and the PACS and RIS would be the engine's transmission of data into actionable reports for clinicians. However, it's essential to ask ourselves if the radiologist will be able to sit behind the driving wheel, and if yes, what will be his function.
Instead of apprehending, ignoring, or refusing this new development, radiologists should rather start using it as a tool for amplifying their services and value. The sooner they embrace this disruption, the more they will be able to guide the creation of applications needed for that purpose.
Looking to the future
Pessimists say that we are drowning in information. We are trying to manage complex problems with complicated solutions. The driving force of today's progress is technology, which is developed by innovators and supported by early adopters. In this digital era we already see new types of AI-related businesses developing in several domains in healthcare.
The practices and use cases are still relatively scarce however, which is mainly due to the cautiousness of healthcare professionals regarding the safety and privacy of patients. In some countries, policy changes are already appearing with more specific demands regarding safe transmission of patient data for research purposes, and regarding the evaluation and validation of diagnostic algorithms.
After a period of initial turmoil, disruptive innovations will improve the quality of healthcare and allow physicians to focus more on direct patient management, communication, and teamwork. Impending U.S. Food and Drug Administration (FDA) approval of first AI-powered diagnostic algorithms will speed up the changes. AI is the new "diagnostic machine" of tomorrow, and we will see more and more of these showcases at the major radiology exhibitions.
Note: An event from the EuSoMII Academy, "Game changers in radiology: A transatlantic overview," will discuss the effect of informatics and AI on medical imaging. It is jointly organized by the European Society of Medical Imaging Informatics (EuSoMII), the Society for Imaging Informatics in Medicine (SIIM), and Erasmus Medical Center. It takes place in Rotterdam, the Netherlands, on 18 November. Click here for more details, or contact Paola Rinaldi at [email protected].
Dr. Sergey Morozov, PhD, is CEO and professor, Research and Practice Center of Medical Radiology in Moscow, and chief radiology officer of Moscow. Dr. Erik Ranschaert, PhD, is chief, radiology department, H. Hart Hospital in Mol, Belgium. Morozov is president and Ranschaert is vice president of the EuSoMII.