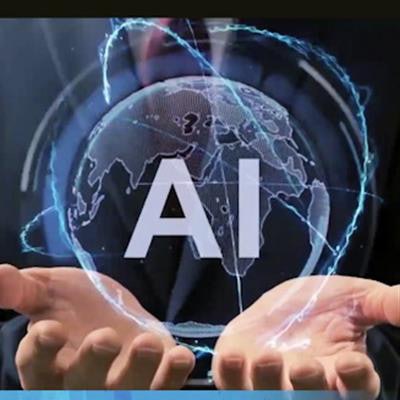
The well-known Belgian radiologist Prof. Paul Parizel has teamed up with a Western Australian colleague to produce an artificial intelligence (AI) primer that is designed to cut through the jargon and the hype.
"It is vital for radiologists to have a thorough understanding of the topic; however, AI is developing so quickly that teaching in many cases has not yet caught up," they noted.
The main aim of the primer is to enable readers to understand the basic concepts of AI and read and talk about AI topics using consistent and accepted definitions. The researchers are keen to boost understanding of how AI will shape the future of radiology and ensure radiologists are familiar with common terminology used when evaluating AI studies.
"I first approached Prof. Parizel about developing the primer after attending his excellent talk at the Western Australia Imaging Workshop in 2020," first author Dr. Phillippa Gray of Sir Charles Gairdner Hospital (SCGH) in Nedlands told AuntMinnieEurope.com. "As a junior doctor and aspiring trainee, I was already doing my own research to educate myself about AI in radiology and I thought it would be an opportunity to consolidate my own learning while also educating others."
Parizel, a past president of the European Society of Radiology who moved from Antwerp, Belgium, in 2019 to become inaugural David Hartley chair of radiology at Royal Perth Hospital and University of Western Australia, and Gray launched the primer in an e-poster presentation at the recent Royal Australian & New Zealand College of Radiology (RANZCR) annual scientific meeting.
Explosion of journal articles
They conducted a literature search of Medline using the key terms "radiology" and "Artificial Intelligence" / "deep learning" / "neural networks" / "machine learning" for all articles published in English in 2019 and 2020. This yielded 1,300 results, from which they selected the top 400 results, based on journal quality (impact factor for 2019). Key areas and examples were taken from the results of this search and distilled into graphical representations of key concepts and examples of recent research to illustrate examples.
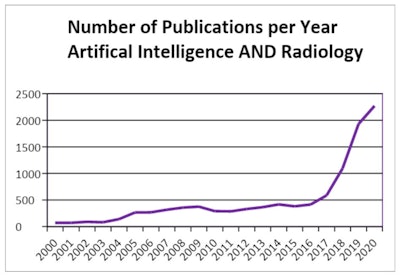
"To understand this new universe of AI, doctors will need to acquire a new language," the researchers explained. "While most doctors are familiar with metrics such as sensitivity and specificity, which are still used when reporting AI research, there are other metrics applicable to computer science, and also some overlap of terms."
For instance, a knowledge of area under curve and receiver operating characteristics (AUC-ROC) is necessary. The curve is derived by plotting the true positive rate (or sensitivity) against the false positive rate (or specificity). It is worth bearing in mind that 0.5 is the worst score for AUC-ROC, because zero would actually mean a tool is perfectly inversely predicting/classifying, they stated.
Referring to 'pixel accuracy,' which is the percentage of pixels in an image that have been classified correctly, the authors noted that while seemingly simple to understand, this term can be misleading and is not commonly used.
The Jaccard Index (or intersection over union) is the area of overlap between the predicted segmentation and the ground truth divided by the area of union between the predicted segmentation and the ground truth. This produces a number between 0 and 1, where 0 = no overlap (worst) and 1 = perfectly overlapping (best). In the dice coefficient (F1 score), the definition of the F1 score depends on whether it is used in a classification task or in a segmentation task.
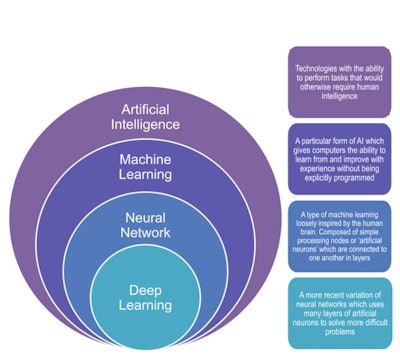
Saliency maps and class activation mapping are also important, according to the authors.
"One of the main issues with deep learning is that it is a 'closed box,' " they pointed out. "The only way to see if it is working correctly is to run the program and manually check that the result is what you are expecting.
"However, it is possible for AI to return seemingly 'correct' results, even with high accuracy, using the wrong data (e.g., using sarcopenia to accurately predict survival instead of tumor characteristics). This is one of the reasons deep learning techniques can require large and varied data sets," they noted.
Often the only way to be able to check on this is with visual representations. Usually depicted in a "heat map" format, this process allows researchers to check that the AI is using the correct area in the image to make its decision.
Applications of AI
Gray and Parizel elaborate on six key areas of potential for AI in imaging:
- Clinical decision support (CDS). Many aspects are being investigated, including aiding with appropriate exam selection, linking patients or healthcare providers with existing CDS tools, or obtaining data to help cast light on the future.
- Image acquisition. Also referred to as "deep-learning reconstruction," deep-learning techniques are being developed to improve technical aspects of image acquisition -- e.g., to reconstruct virtual high-dose CT images from low-dose CT images with reduced metal artifact.
- Image processing. Automating and refining aspects of image processing that should be more accurate and less time-consuming to assign to machines, such as measuring lesion or organ volume or counting large numbers of lesions (e.g., nodules or metastases).
- Augmented detection. Probably the most talked about use of AI in radiology, where the AI either flags areas it thinks contain pathology or ultimately acts as a first or second reader. This may be useful in areas where there are reduced numbers of radiologists, e.g., a neural network trained to identify meniscal tears on knee MRI scans.
- Disease classification/outcomes. Referred to as radiomics, this is often compared with biomarkers, where image features that predict disease (but are not delectable to human vision) are identified using deep learning techniques. Another classification system is called morphomics, which involves looking into patient data collected at the time of scanning for prognostic information.
- Reporting. There are many ways reporting can benefit from AI, from autogenerating information obtained in the image processing or disease classification stage to generating entire reports. Development of a machine-learning model can identify a follow-up recommendation in free-text reports, which can be used to highlight or link such a recommendation to providers and reduce delay in follow-up.
Gray presented a second e-poster at the ASM called, "Current examples of segmentation techniques for medical imaging analysis." Gray was recently accepted onto the radiation oncology training program and is keen to continue her research in segmentation/contouring, specifically. Gray has already been involved in a joint project between the radiology and radiation oncology departments at SCGH to acquire amide proton transfer (APT) MRI.
Editor's note: You can view the AI primer by going to the link to the full poster on the RANZCR section of the Electronic Presentation Online System (EPOS) on the European Society of Radiology's website. At the time of submission, the research was done under the affiliation of the dept of radiology at Royal Perth Hospital (RPH).