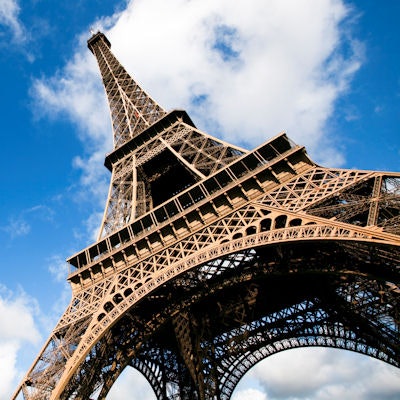
An artificial intelligence (AI) algorithm can accurately predict billing codes from log data of MRI studies, potentially helping to avoid coding errors and reducing the workload of radiographers, German researchers told attendees at the annual meeting of the International Society for Magnetic Resonance in Medicine (ISMRM 2018) in Paris.
In a proof-of-concept study, researchers led by Jonas Denck of the University of Erlangen-Nuremberg in Erlangen trained a machine-learning algorithm that can determine the correct billing codes for a MRI exam with over 97% accuracy -- based just on reviewing the study's log data.
"The results show the potential of a machine-learning application for the task of automated billing for MRI exams, with a performance that is close to the human performance," the authors noted in a poster presentation at ISMRM 2018.
A cost-intensive modality
MRI is a time-consuming and cost-intensive modality, and it's crucial to correctly report billing codes in order to properly claim the incurred costs for these studies, according to the researchers. However, the current approach -- manual entry of procedures and associated billing codes by a technologist -- can be prone to errors, leading either to missed or wrong codes.
As a result, the researchers sought to develop an algorithm that could automatically provide billing codes from data included in MRI logs, which contain information such as executed sequences, scanner table movement, and registered body region, etc. In the first step of the training process, features were extracted from the MRI sequences, including a subset of sequence parameters, MRI scanner table movements, anonymized patient information (age, gender), the registered body region, and information on whether contrast was used in the study, according to the researchers.
In the second phase, normalized sequence names were automatically generated based on their underlying parameters, which made the algorithm easier to train and more generalizable, according to the researchers. A feed-forward neural network with a single hidden layer was then trained from actual study billing codes -- for the TarMed outpatient fee-for-service tariff system in Switzerland -- that were extracted from the RIS at a single hospital.
The researchers developed the algorithm using a training set of 7,972 MRI exams and a test set of 448 MRI studies, which were acquired from either Magnetom Aera or Skyra scanners (Siemens Healthineers) at the institution. After data cleaning, there were 22 billing codes, with an average of 4.1 billing codes assigned for each MRI exam. Manual billing errors were observed in some of the test cases, so the researchers made corrections in order to generate a ground-truth test dataset.
Accurate predictions
As a result, the researchers were able to compare the performance of the algorithm's automated predictions with the billing codes entered manually by the technologist. Accuracy was calculated on the test set using the F1 score, as shown the table below.
Performance for identification of correct MRI billing codes | ||
Manual entry of billing codes | Machine-learning algorithm | |
Micro-averaged F1 score | 98% | 97.1% |
Precision | 98.7% | 96.9% |
Recall | 97.4% | 97.3% |
While manual billing was still superior to the algorithm, the researchers noted that neither manual billing nor the algorithm produced the correct set of billing codes in 3.7% of the cases. What's more, manual billing was better than the algorithm in 8.3% of the test cases, while the prediction outperformed the manual method in 4.3% of the cases.
"Thus, the prediction outperformed manual billing for a significant share of the test instances, although the predictive accuracy was still lower," Denck pointed out, who last year completed his master's thesis in medical engineering about automatic billing code retrieval from MR scanner data.
The researchers noted MRI log data also has limitations, including gaps due to logging errors. In addition, log data also lacks information, for example, on the type and amount of injected contrast agent. However, the machine-learning algorithm could potentially also be applied to similar datasets such as DICOM headers, they said.
Nonetheless, MRI billing procedures need to be improved in order to reduce errors, according to the researchers.
"Integrated in the clinical environment, this work has the potential to reduce the workload for technologists, prevent coding errors, and enable scanner-specific expense and turnover analysis," wrote the authors, which included Wilfried Landschütz of Siemens; Dr. Knud Nairz and Dr. Johannes Heverhagen, PhD, of the University Hospital of Bern in Switzerland; Andreas Maier, PhD, of the University of Erlangen-Nuremberg; and Eva Rothgang, PhD, of the Technical University of Applied Sciences Amberg-Weiden in Amberg, Germany.