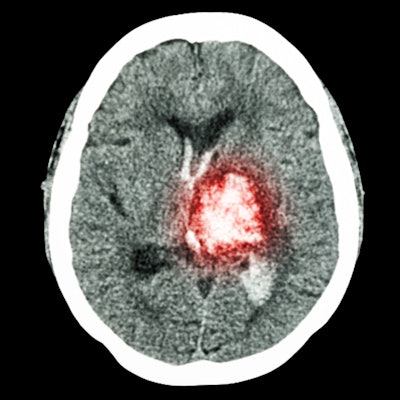
An AI prediction model that incorporates CT radiomics features and clinical information can significantly improve outcome predictions in patients with acute ischemic stroke, according to a study published on 3 July in the European Journal of Radiology.
Researchers led by first author Limin Zhang and corresponding author Dr. Wei Zhang of the Second Affiliated Hospital of Chongqing Medical University developed three machine-learning prediction models based on radiomics from noncontrast CT exams, clinical features, and a combination of the two. The combined model yielded the best performance, delivering nearly 94% sensitivity and 85% accuracy.
"... a fusion model that integrates clinical and radiomics data, utilizing easily accessible, efficient and noninvasive [noncontrast CT] imaging, has the potential to predict the clinical outcomes of [acute ischemic stroke] patients receiving conventional treatment, which can help guide early clinical intervention to improve their outcomes in time," the authors wrote.
To test their hypothesis that machine learning-based radiomics models could add value in predicting functional outcome at six months, the researchers first mixed 197 patients from one hospital and 43 patients from a second hospital to form a training cohort of 194 cases and a testing cohort of 46 patients.
Two junior radiologists utilized the open-source 3D Slicer software to manually segment the infarct lesions on the noncontrast CT scans. Seven radiomics features were then extracted from the images.
Next, the researchers trained three different machine-learning prediction models to construct the prediction models, including a radiomics model as well as a clinical model based on clinical data. The combined model used input from both the clinical features model and the radiomics model. All models were trained to predict the modified Rankin scale six months after hospital discharge.
On the test set, the combined model produced the highest area under the curve (AUC), sensitivity, and accuracy, but it was also the lowest in specificity.
Performance on test set for predicting outcomes in acute ischemic stroke patients | |||
Clinical model | Radiomics model | Radiomics + clinical model | |
Area under the curve | 0.643 | 0.705 | 0.857 |
Sensitivity | 65.6% | 81.2% | 93.8% |
Specificity | 78.6% | 78.6% | 64.3% |
Accuracy | 69.6% | 80.4% | 84.8% |
"Given the encouraging results of this study, larger independent data sets and more sophisticated machine-learning algorithms will make image analysis based on [noncontrast CT] more promising in the future," the authors wrote.
The full journal article can be found here.